The State of Adopting AI in Drug Discovery in 2025
AI adoption is growing increasingly more prevalent globally, including in the drug discovery space. While this has huge potential, real-world usage is limited by issues like AI models hallucinating, having a strong confirmation bias and the necessity of entrusting these models with sensitive data.
With the help of Adrian Stevens and Jan Christopherson we wanted to take a snapshot of the drug discovery industry’s current AI usage trends. They recently attended the Bio-IT World 2025 and authored this blog post together to account their takeaways.
Keep reading for a review of where the industry stands with respect to implementing AI in 2025.
Chemaxon team from left to right: Adrian Stevens (CPO), Richard Jones (CEO), Jan Christopherson (Senior Application Scientist)
State of AI in drug discovery in previous years
We have just waved goodbye to another Bio-IT World conference in Boston, Massachusetts.
It was again heavily dominated by topics around the applications of Artificial Intelligence and Machine Learning in drug discovery. In past years, depending on the presenter, the talks covering AI have often swung wildly between either heavily skeptical or high optimism verging on hyperbole. Hence, neither of us were quite sure how these topics would fare in 2025.
AI in drug discovery in 2025
This year, there was evidence of a marked change in the AI-based talks.
Significantly, while the opposing skeptic or optimism undertones were still present, it was evident that the presenters were making serious efforts to find meaningful applications for artificial intelligence. From the keynote kickoff session through to the panels and presentations on the final day, three key messages could be recognized throughout.
The key themes of using AI for drug discovery in 2025 were:
- the democratisation of the use and creation of AI models,
- the creation of central corporate guardrails,
- the need for transparency and explainability of AI model outputs.
AI agents in drug discovery
There was a significant uptick in the number of presenters speaking about how they have enabled scientists and data scientists to interface further with generative AI assistants. The following workflows seem to have been impacted most:
- assistance in writing documents,
- easing the creation of POC applications,
- finding & querying data.
The final point is especially interesting, as it appears Large Language Models (LLMs) may have significantly eased the difficulty with which teams find information across the data silos that exist across their companies, and reduced the barrier to FAIR data. In many of the presentations, RAG (Retrieval-Augmented Generation) was used significantly to improve the performance of the AI models.
AI in drug discovery example by Certara
Our own Sean McGee spoke about the offerings from Certara, focusing on how AI can augment the work of researchers.
The key focus was on closing the data loop in the DMTA (Design-Make-Test-Analyze) cycle, our future outlooks on extracting chemistry from unstructured data, and using systems like D360 as a query and visualization tool for GPT explainability.
AI in drug discovery example by Novartis
Representing the Pharma industry, possibly a great example of the outbreak of real-world pragmatism to application of LLMs was evidenced in the talk by Rishi Gupta from Novartis.
He set the scene of the plethora of internal reports and presentations in Novartis as an untapped goldmine of accumulated knowledge of the company's scientists. In their document form, these are simply not practical to search and reference. However, used as inputs for RAG-based LLMs, their value became apparent.
Enforcing rules for AI usage in drug discovery
The concerns around AI regarding data privacy and the potential damage by inappropriate use or access must be addressed.
As adoption of AI models, specifically large language models and agentic workflows is spreading, it became clear that the creation of centralized guardrails are becoming unavoidable. Some of these practices have been proving successful in moving us away from the wild west of yesteryear.
The following practices seem to be most impactful:
- on-premise deployment of LLMs,
- the creation of risk profiles dictating the permitted level of machine prediction involvement in a decision,
- the validation of specific models for high risk tasks.
Increasing the transparency of AI
The transparency of the model is essential in creating traceability and trust for the scientists leveraging it. Links to papers or internal data sources, queries that show corroborating data, & most similar small molecule structures in training sets are core examples of this.
Summary
There was an interesting comparison between the adoption of AI and the adoption of cloud technology. Both were brewing for a long time, then exploded seemingly in a short time window.
The message is to embrace the adoption of both. And remember: the cloud augments data centers and does not wholly replace them, just as AI augments the work of the scientists but does not replace them.
Was this article useful?
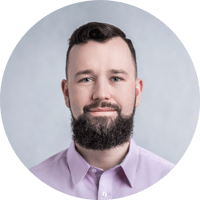
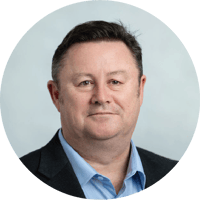