About ChemTalks
ChemTalks is a step up from decades of User Group Meetings to a fully-fledged annual conference. The first ever ChemTalks brought together renowned industry experts, our customers and the Chemaxon team to discuss how technology can bridge silos in early drug discovery. Meet our speakers, discover their topics and browse event photos below.
Speakers and talks
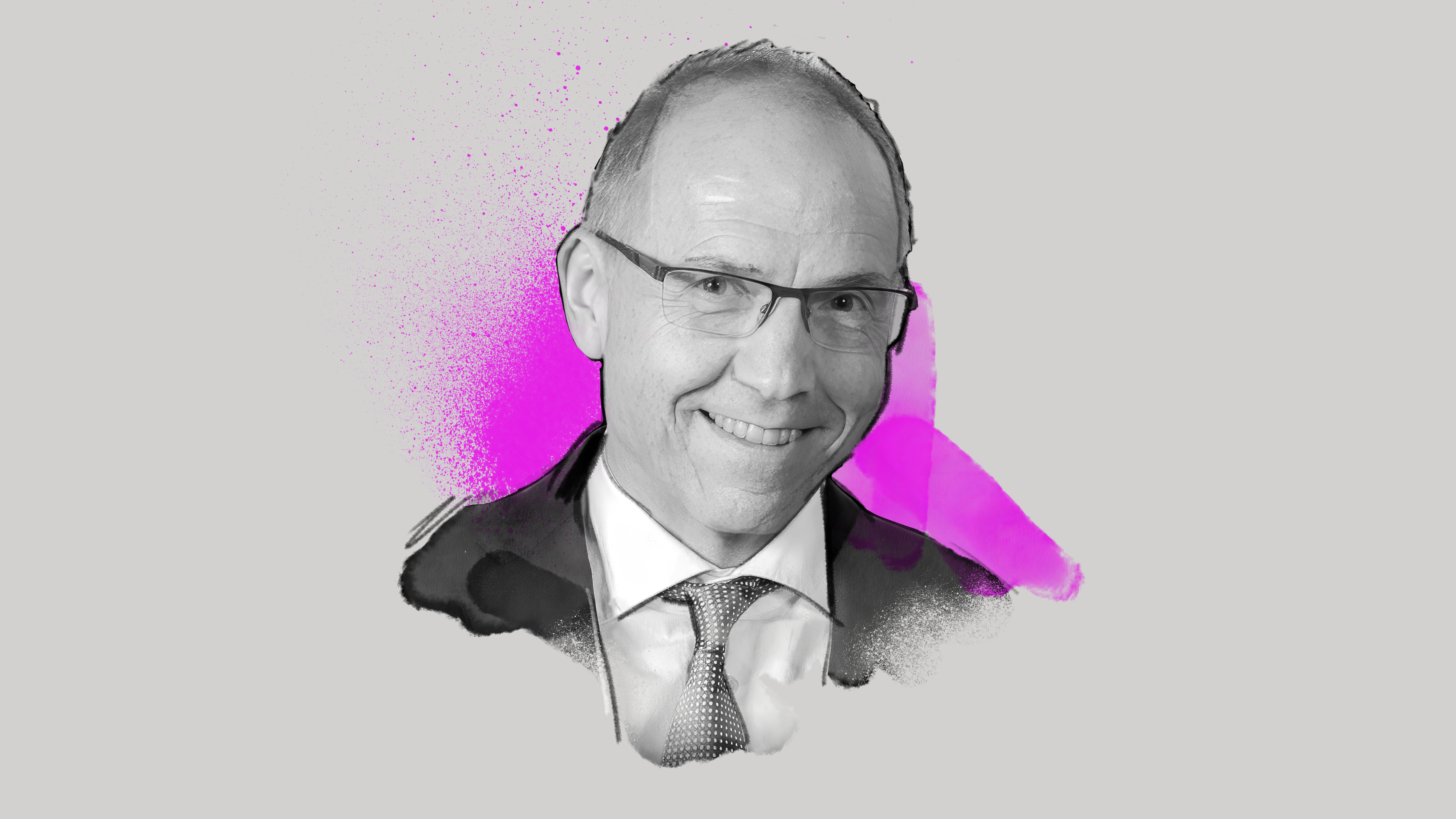
Karl-Heinz Baringhaus
Sanofi
Grasping opportunities for better drug discovery: Interdisciplinarity, deeper insights into disease, new technologies and better decision-making
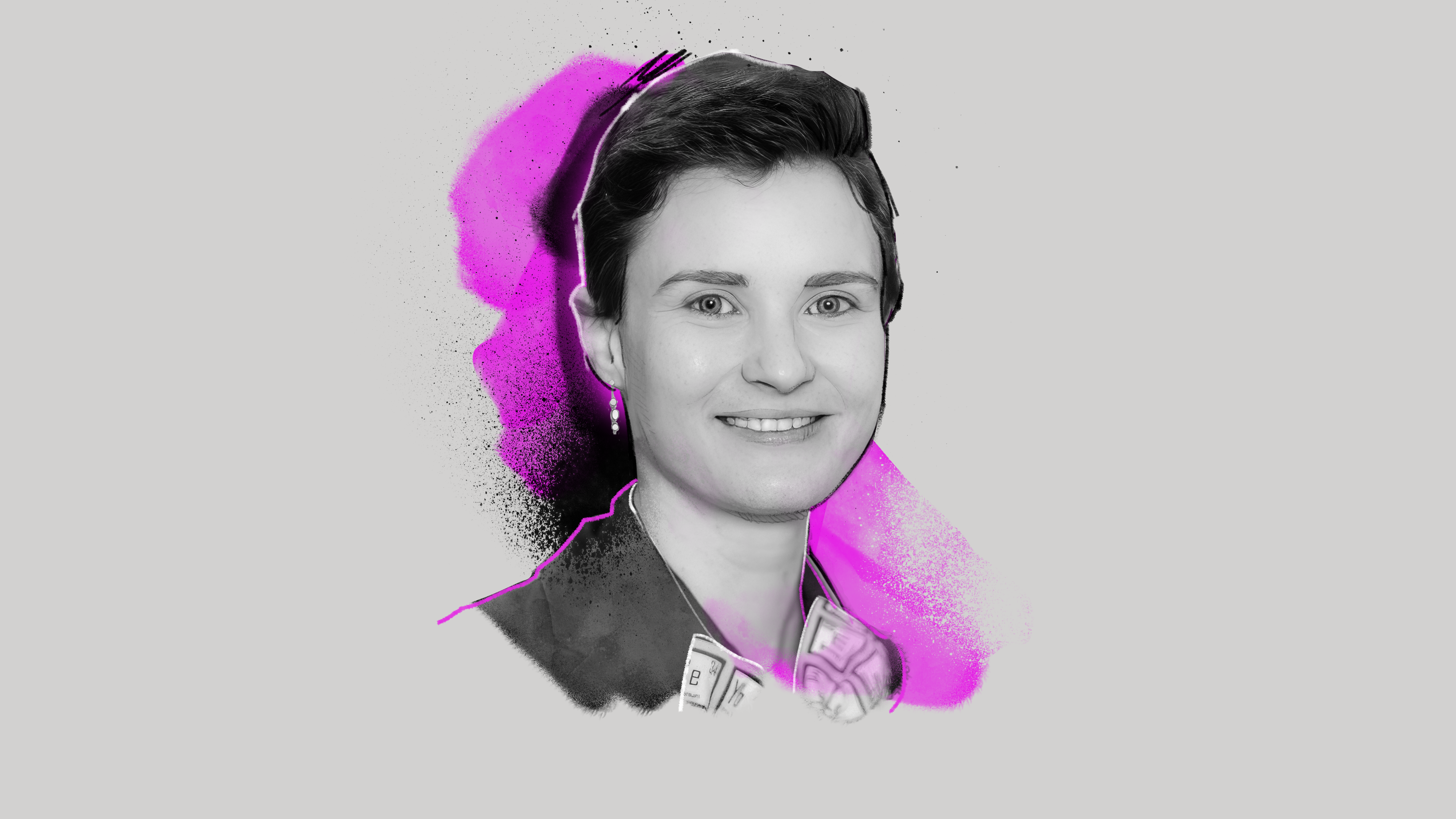
Nessa Carson
AstraZeneca
How not to waste a chemist's time: Chemical insights through great user experience
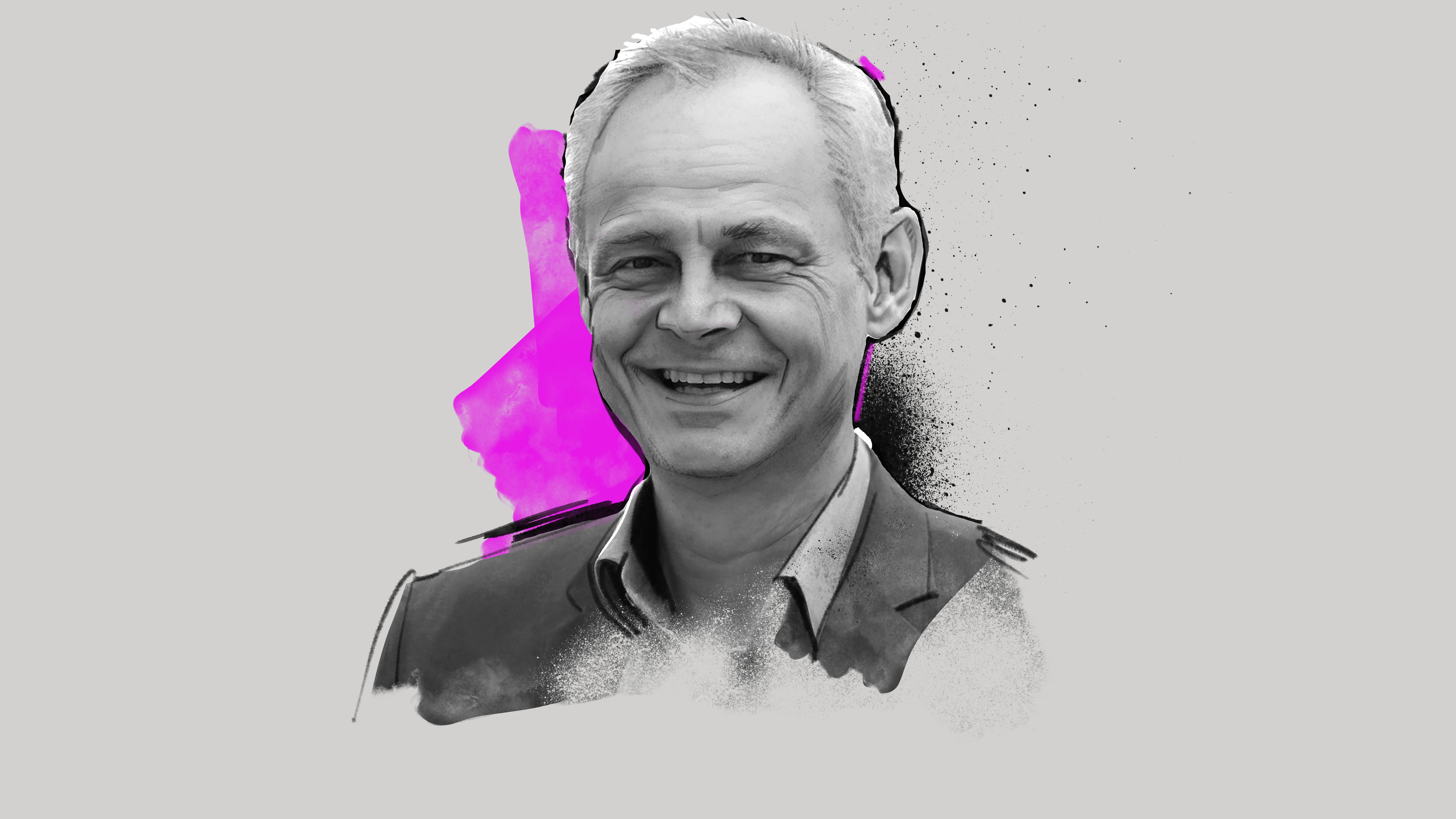
Josef Eiblmaier
PharmaLex, a Cencora company
The beauty of heterogeneity versus the need for standardization: Enabling trusted data consumption in a multi-source, multi-ownership data landscape
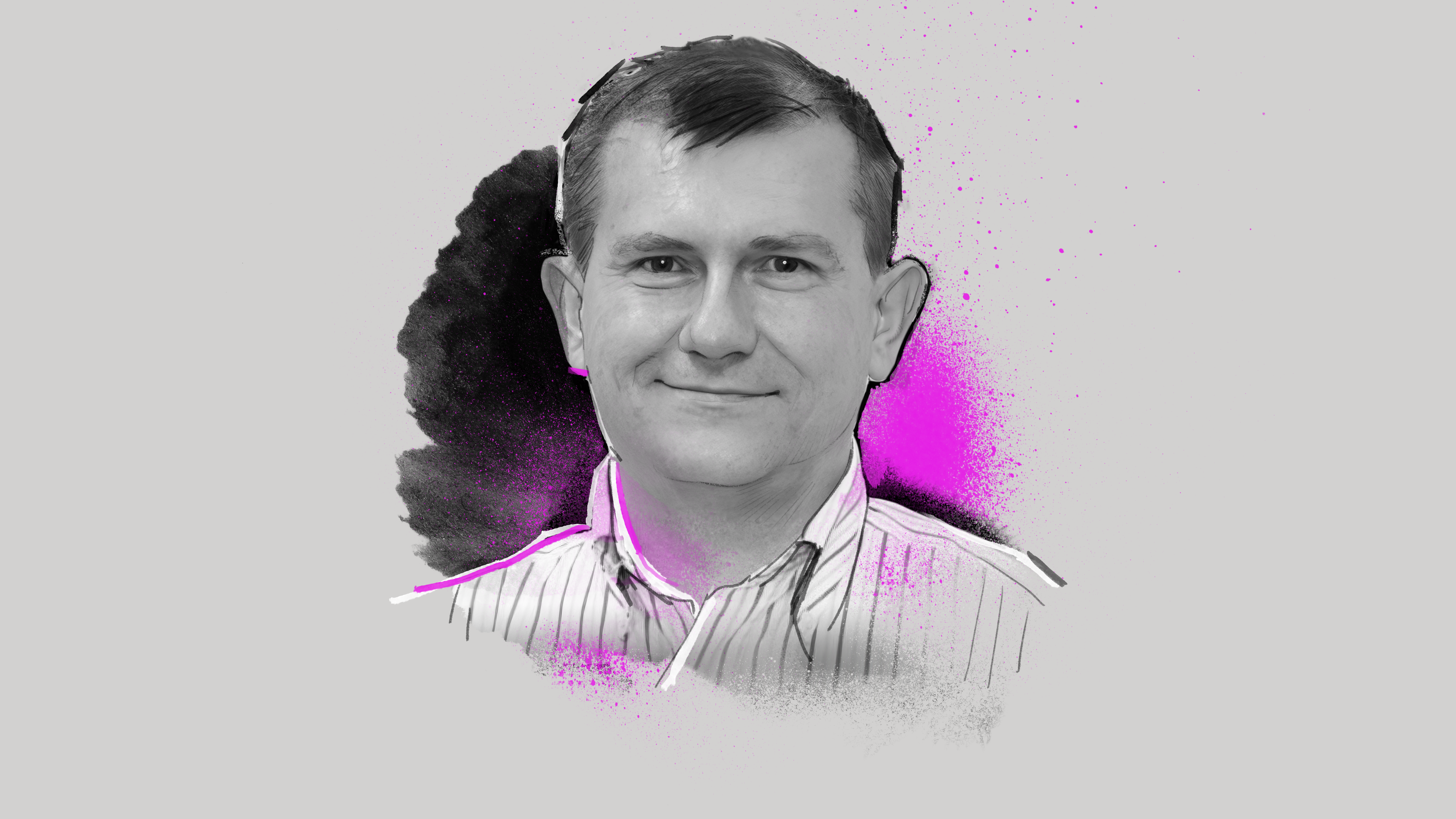
Peter Ertl
Formerly of Novartis
The magic rings: Navigation in the ring chemical space guided by the bioactive rings
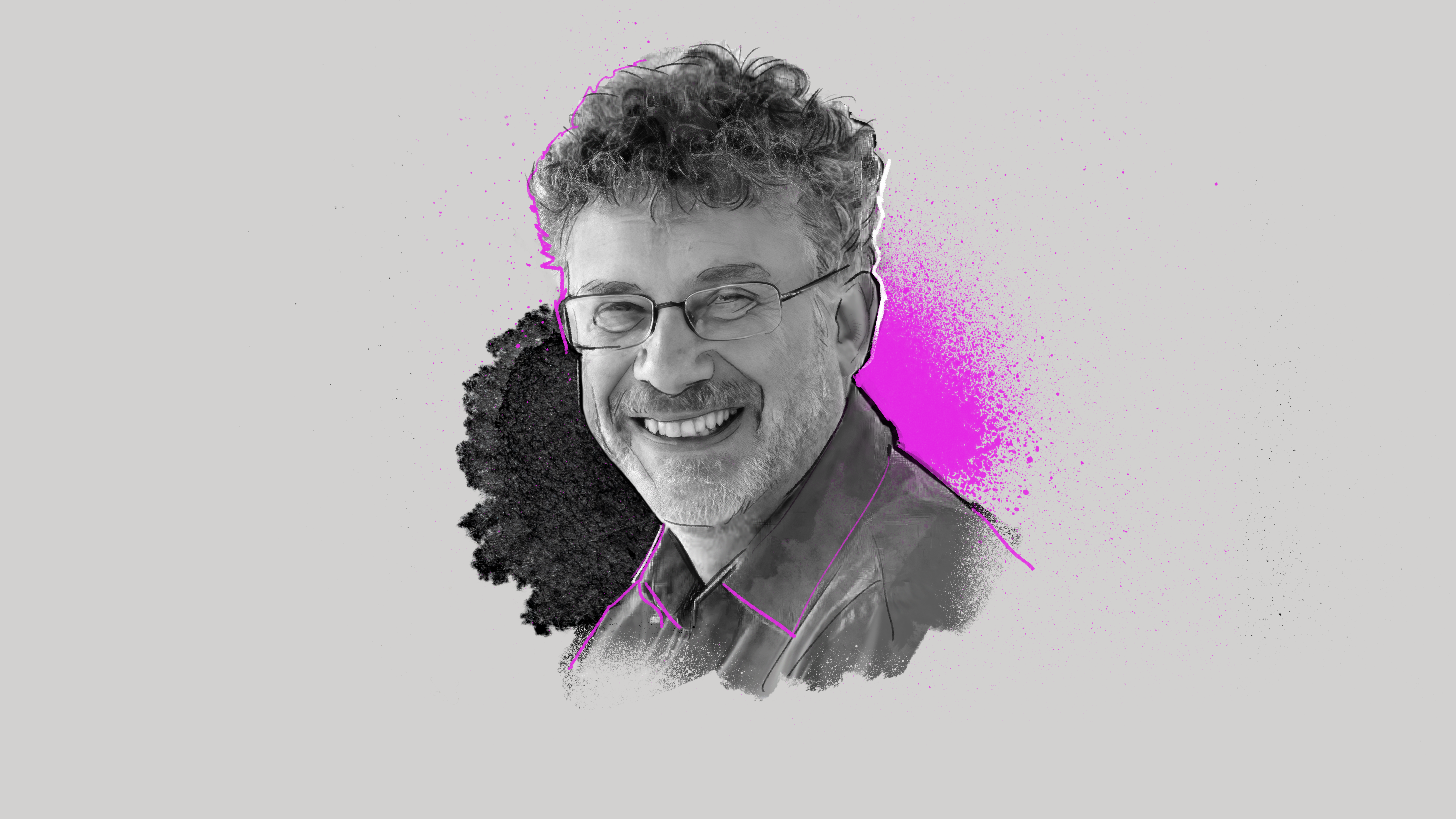
Jeremy Frey
University of Southampton
Digital chemistry: Paradigm shift, cultural evolution or business as usual?
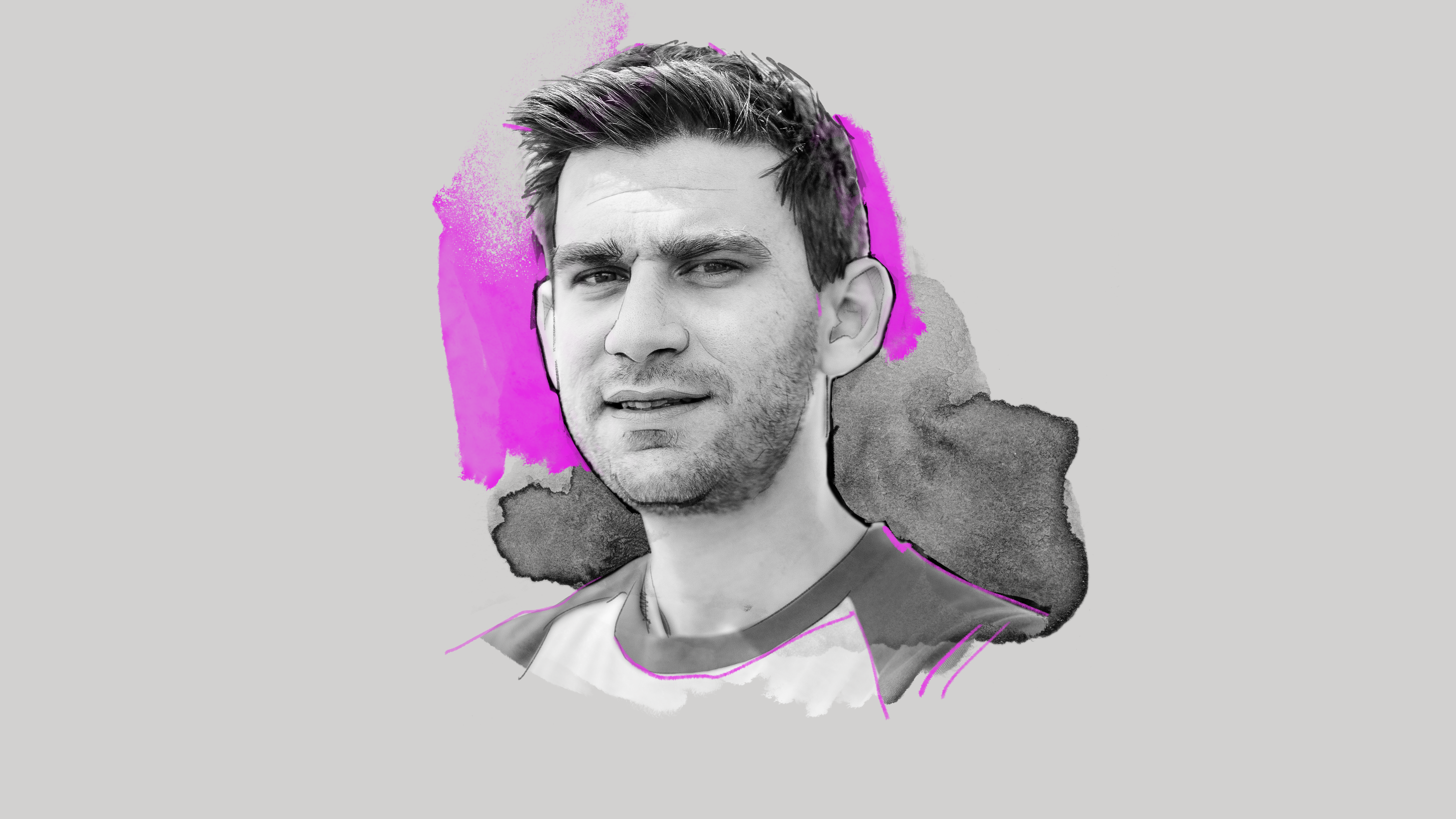
Thrasyvoulos Karydis
Deepcure
Is AI in Drug Discovery trapped in the data of the past?
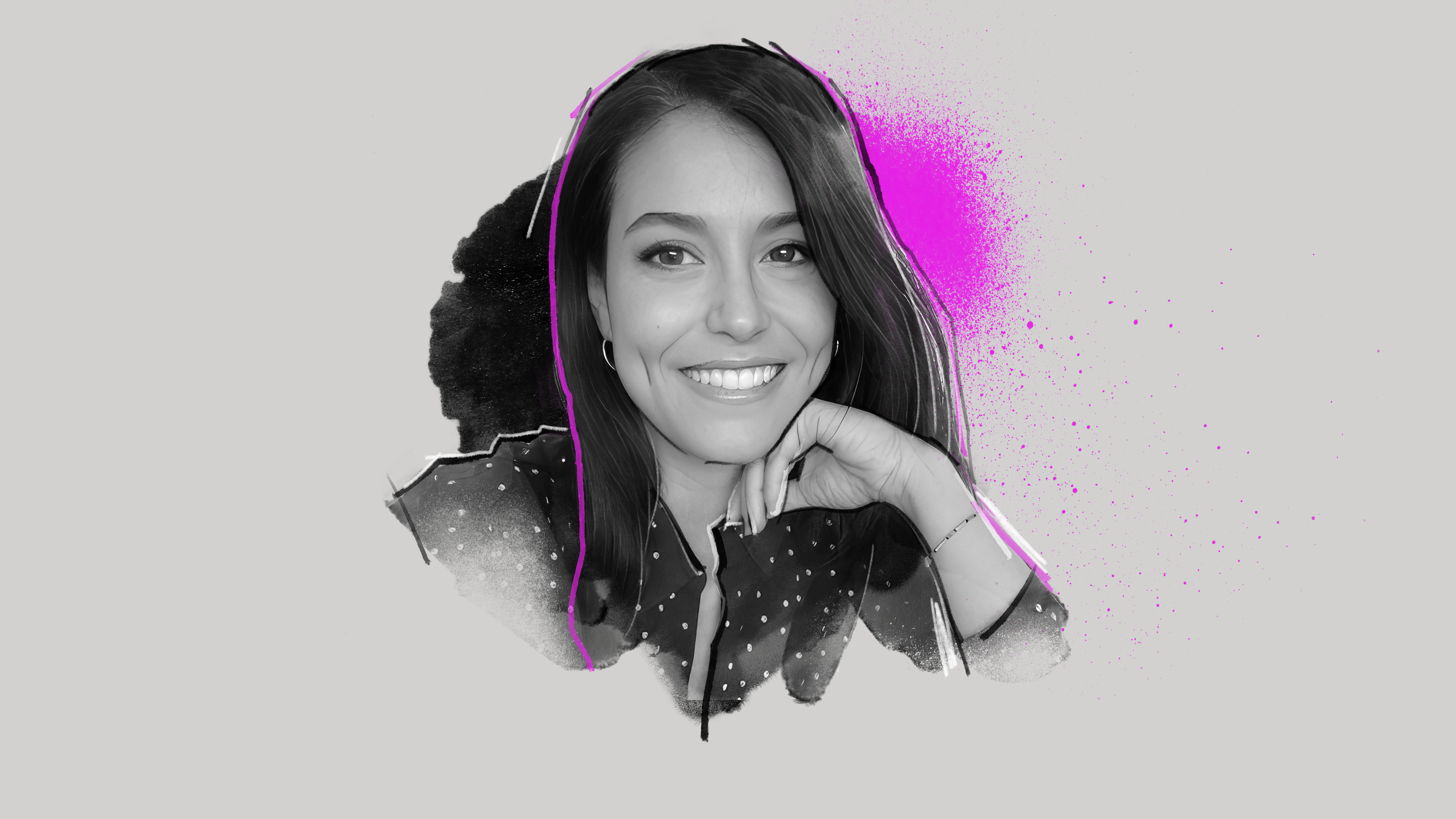
Jessica Lanini
Biomedical Research, Novartis
AI advancing drug discovery research in the pharmaceutical industry and academia
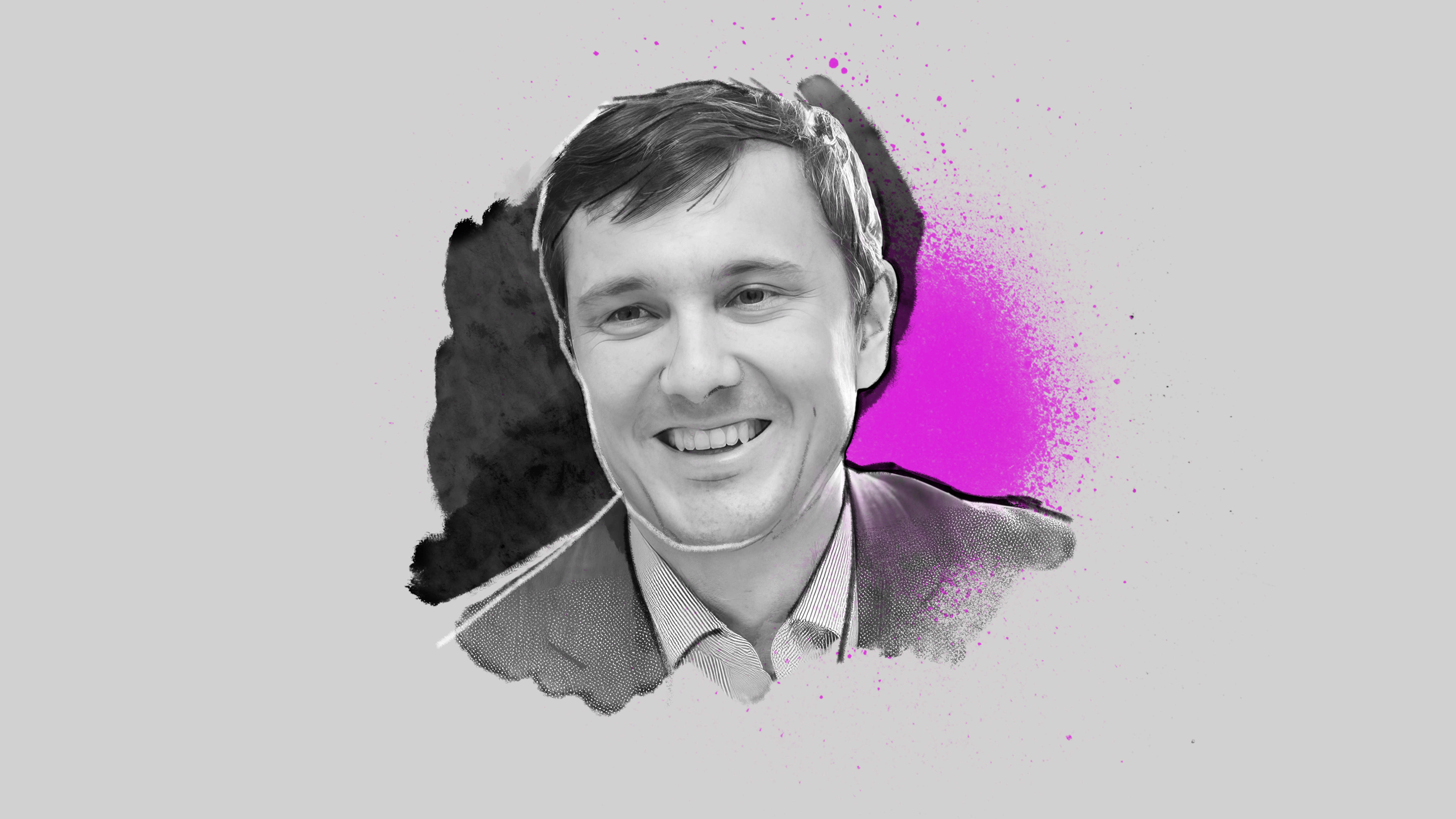
Timur Madzhidov
Elsevier
Unlocking the power of data from disparate sources – Elsevier’s journey toward accurate reaction outcome predictions.
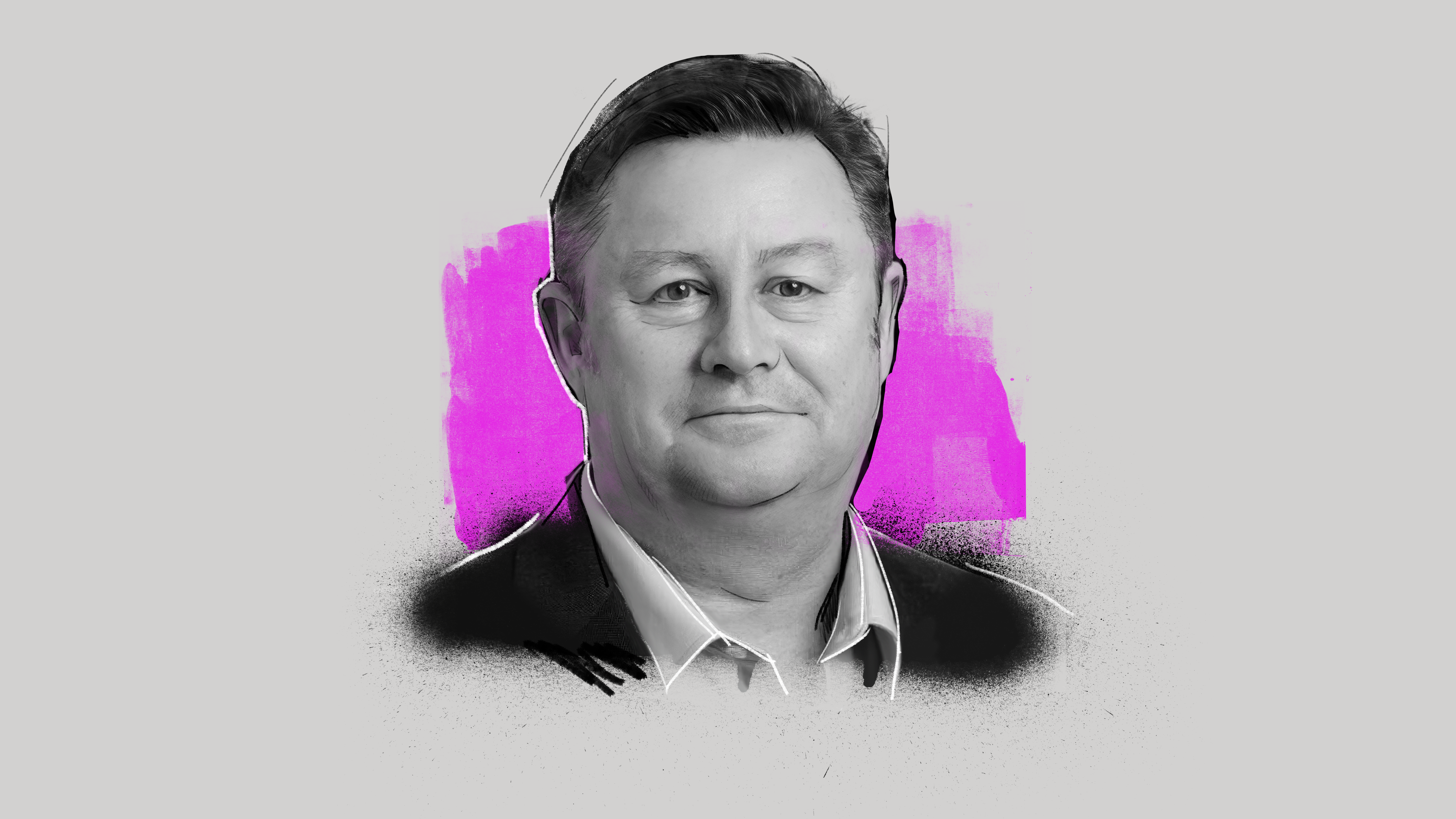
Adrian Stevens
Chemaxon
Developing new science and technologies that aid future drug discovery needs
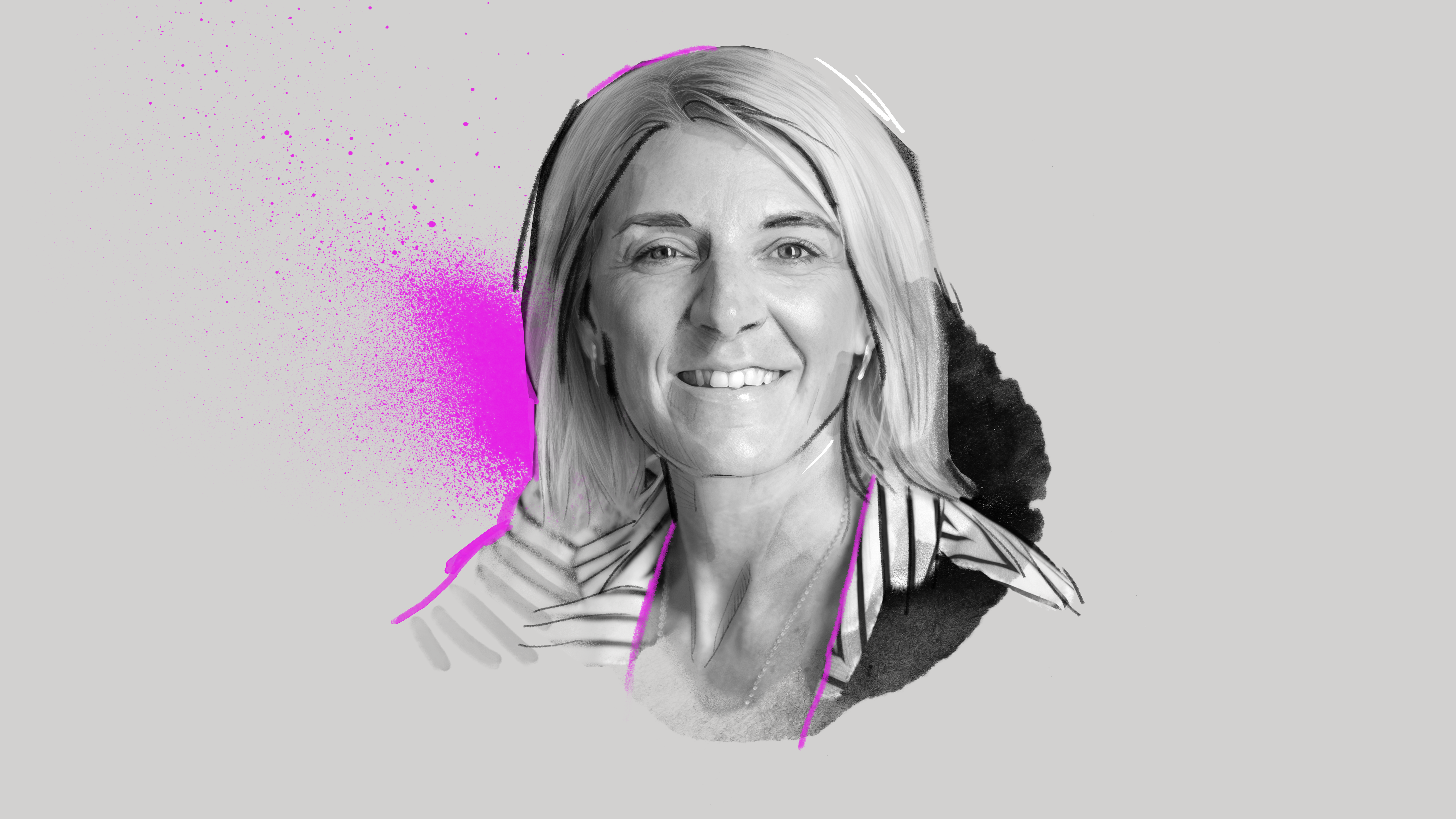
Becky Upton
Pistoia Alliance
Ontologies in Pharma: The landscape, pre-competitive development, and use-cases
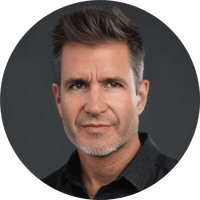
“I enjoyed the ChemTalks conference a lot. The talks were great and Chemaxon excelled at being a wonderful host.”
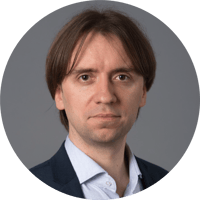
Oleksandr Levenets
Program Director, Medicinal Chemistry and AI, Oncodesign Precision Medicine
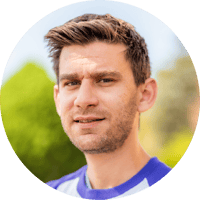
Thras Karydis
Co-founder & CTO, DeepCure
“The conference was one of the best I've been in the cheminformatics space, with a good blend of stimulating industry and academia talks.”
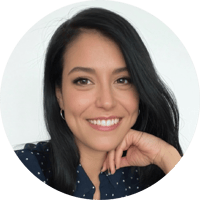
Jessica Lanini
Senior Data Scientist, Novartis, Biomedical Research
Photo Gallery
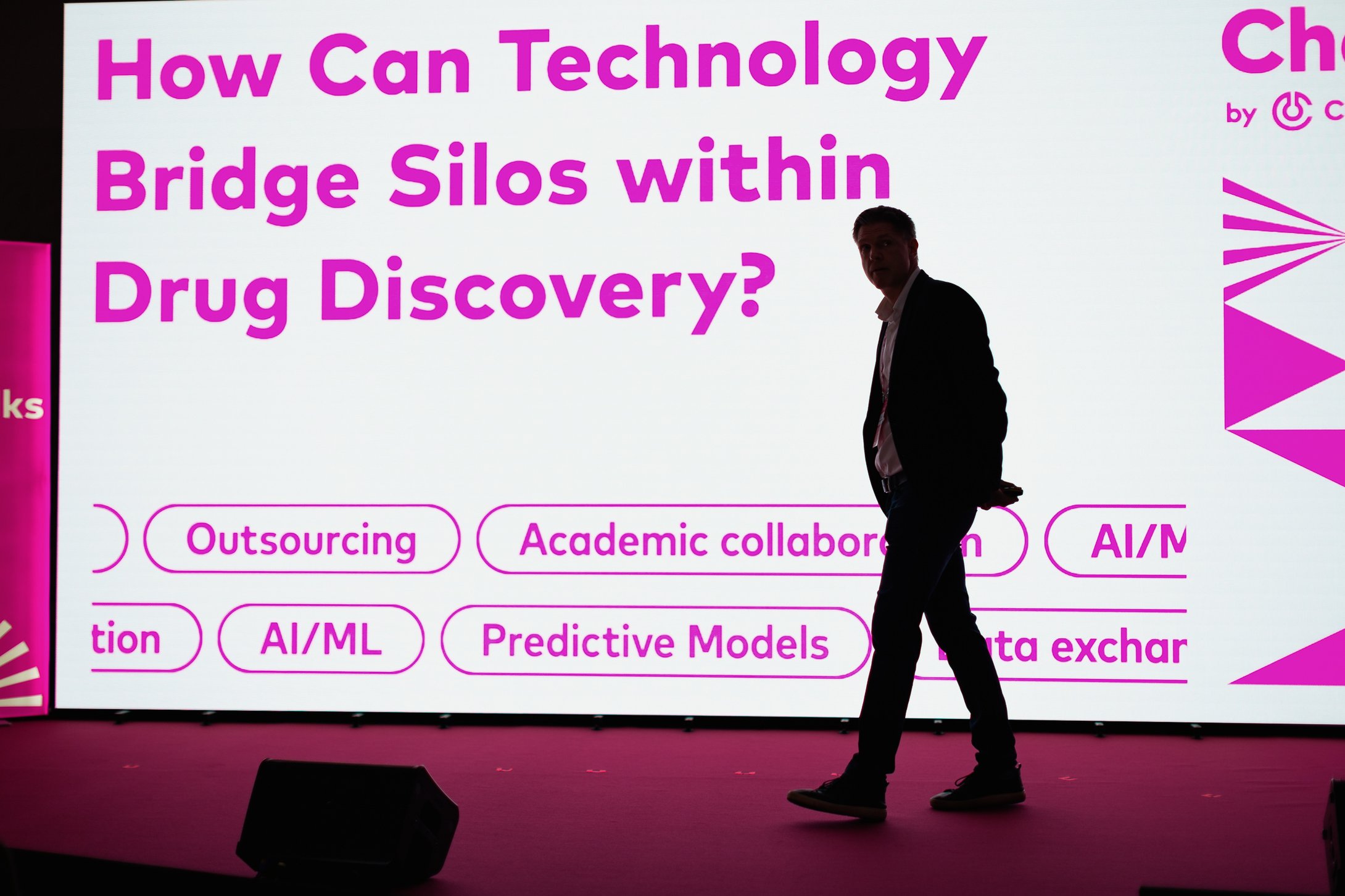
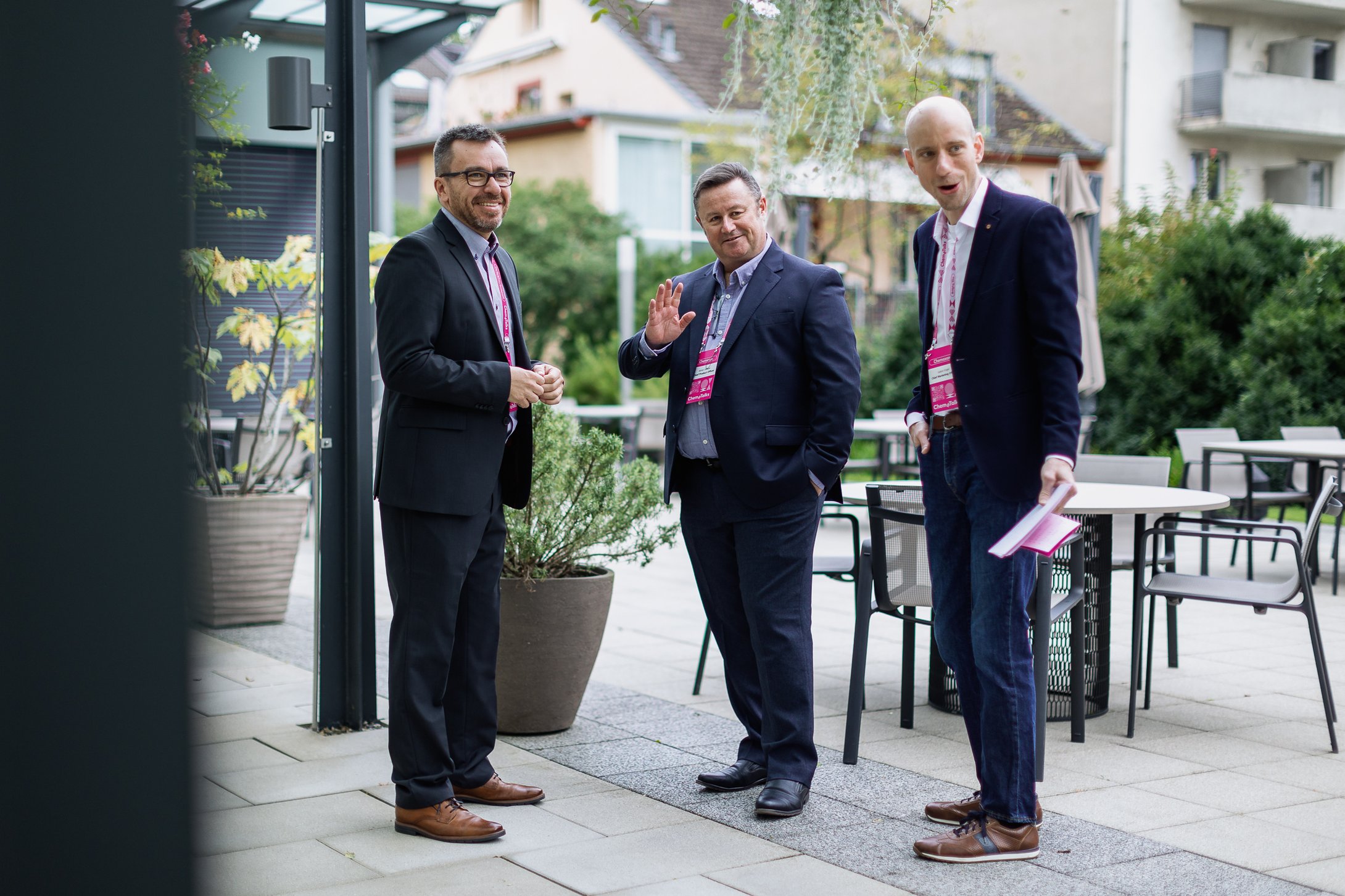
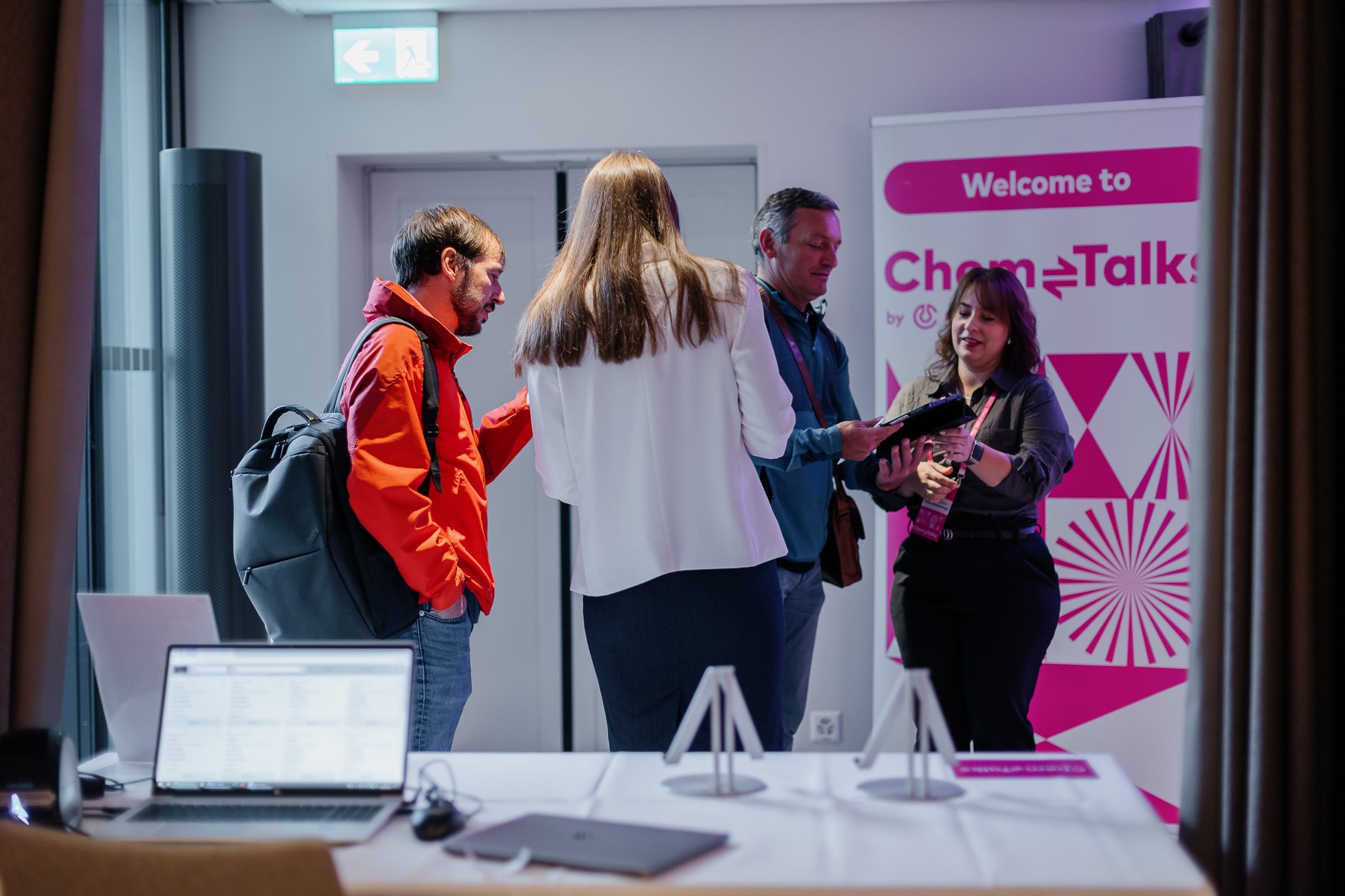
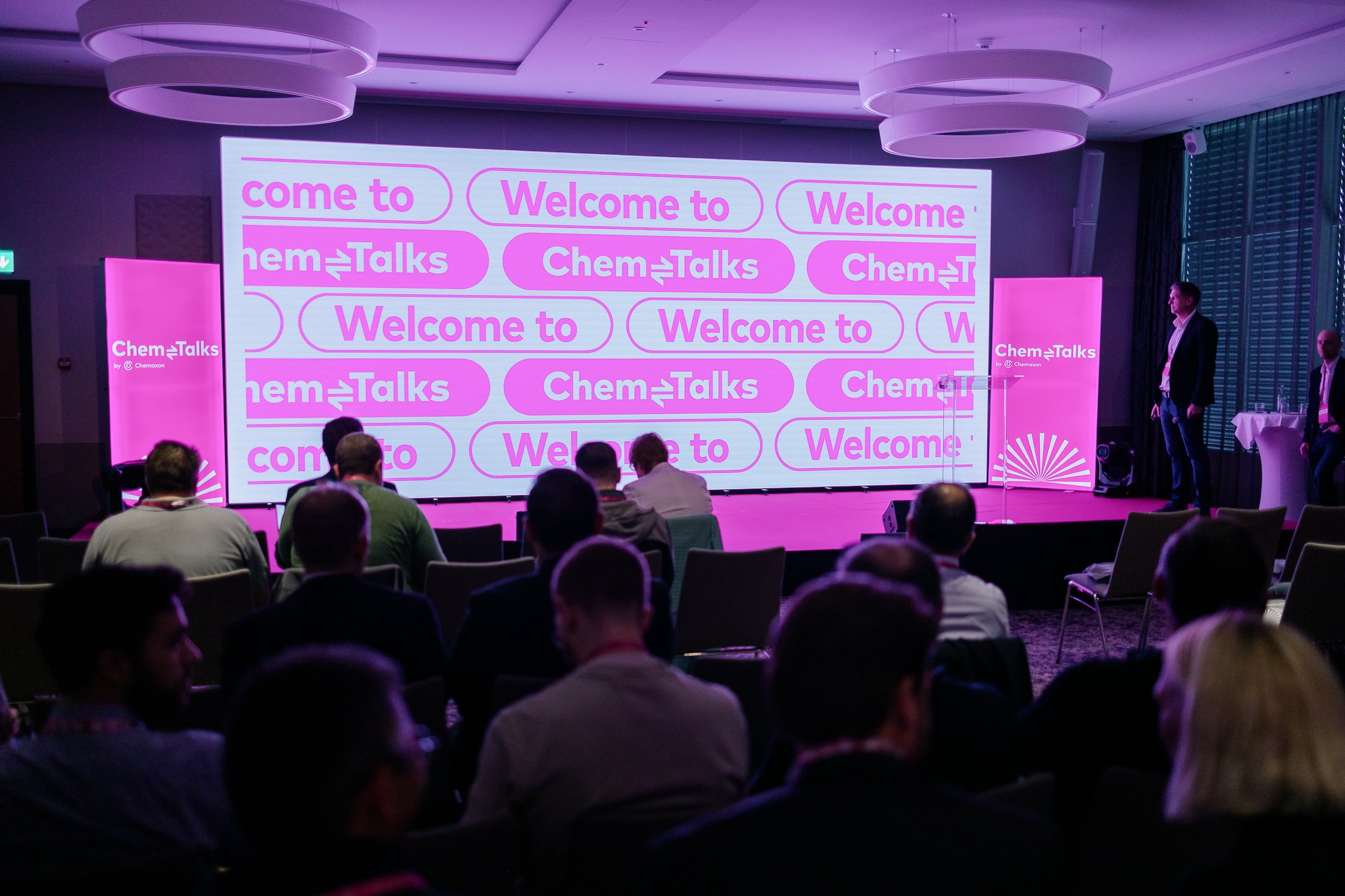
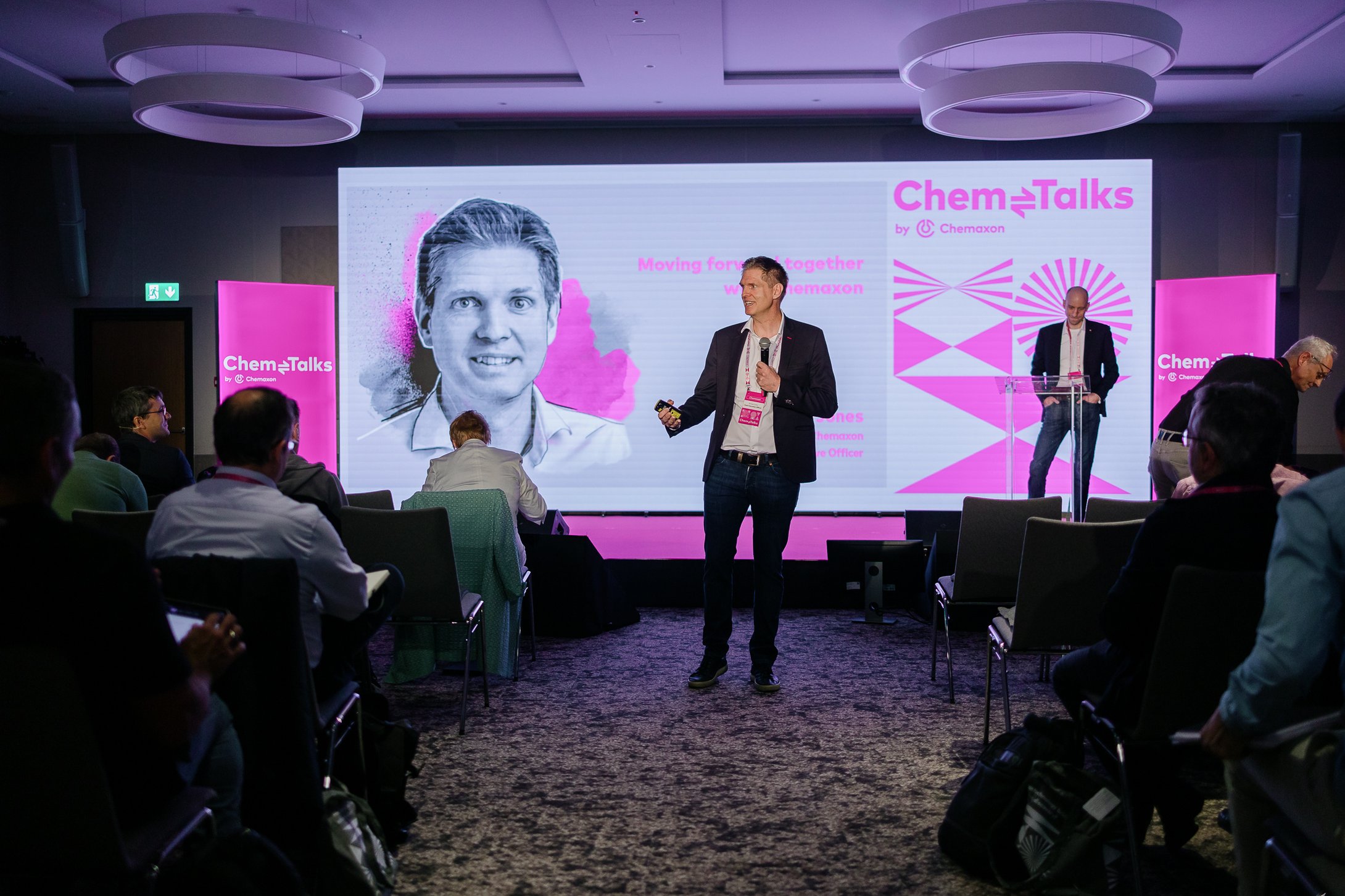
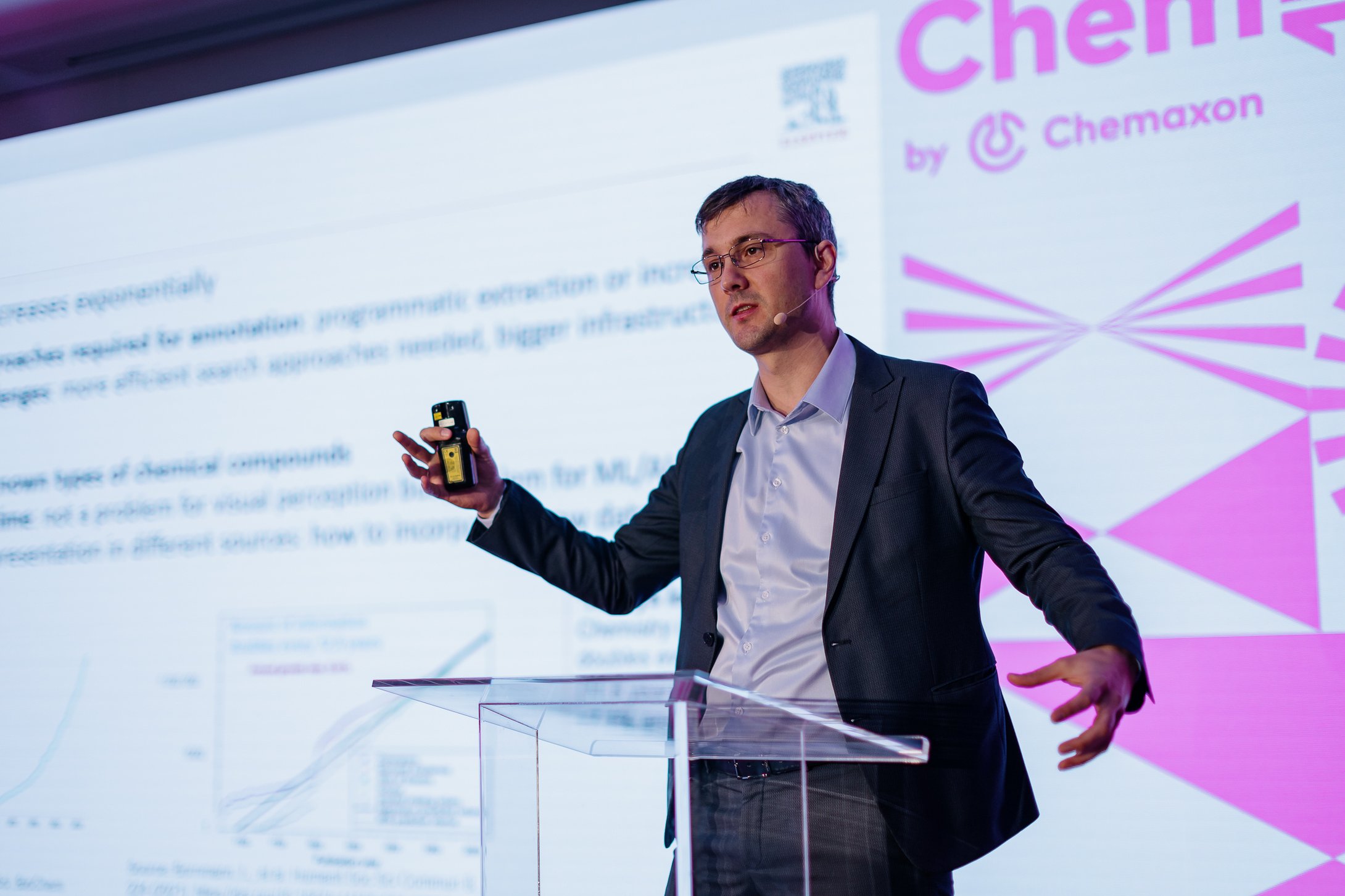
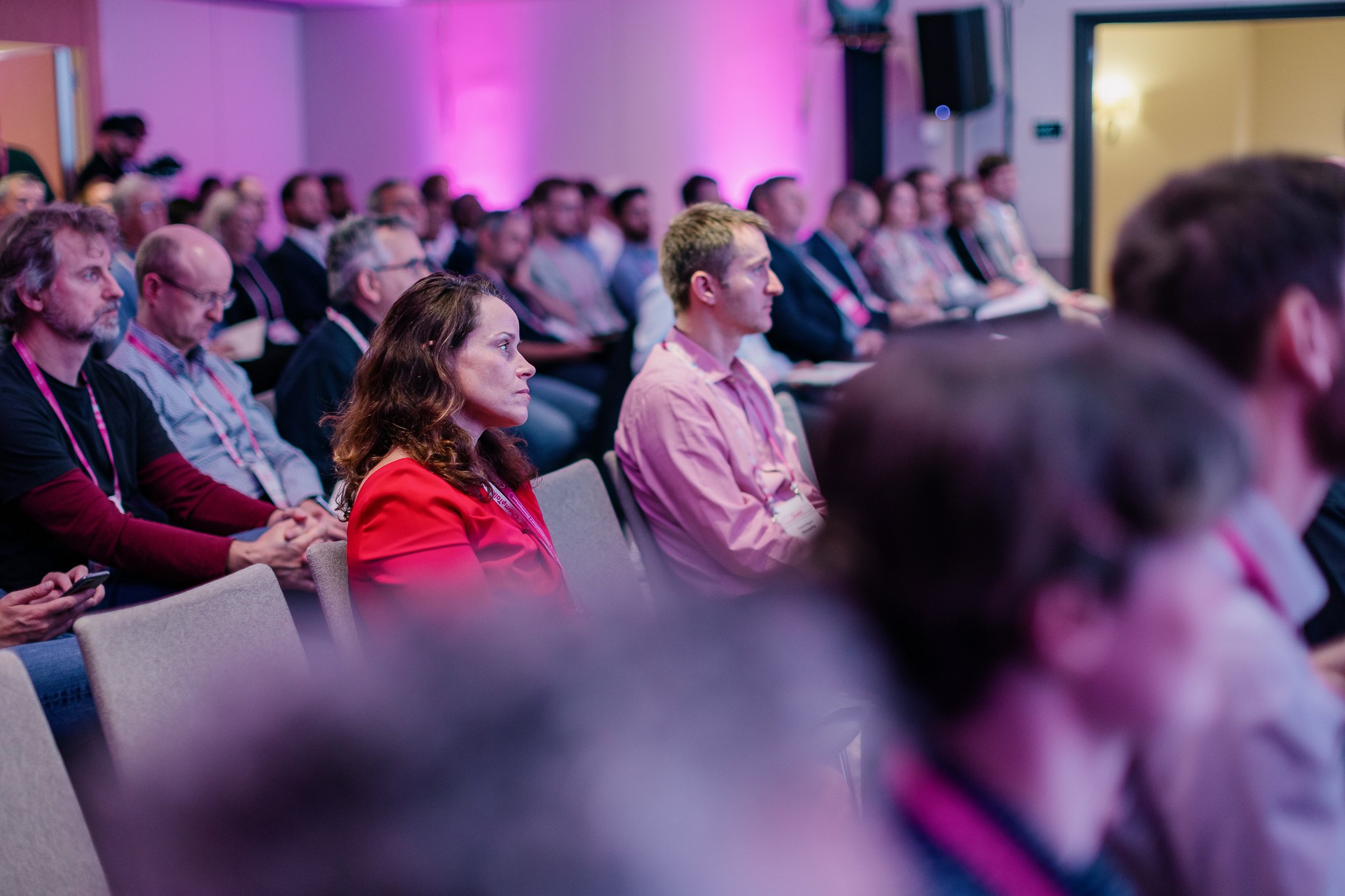
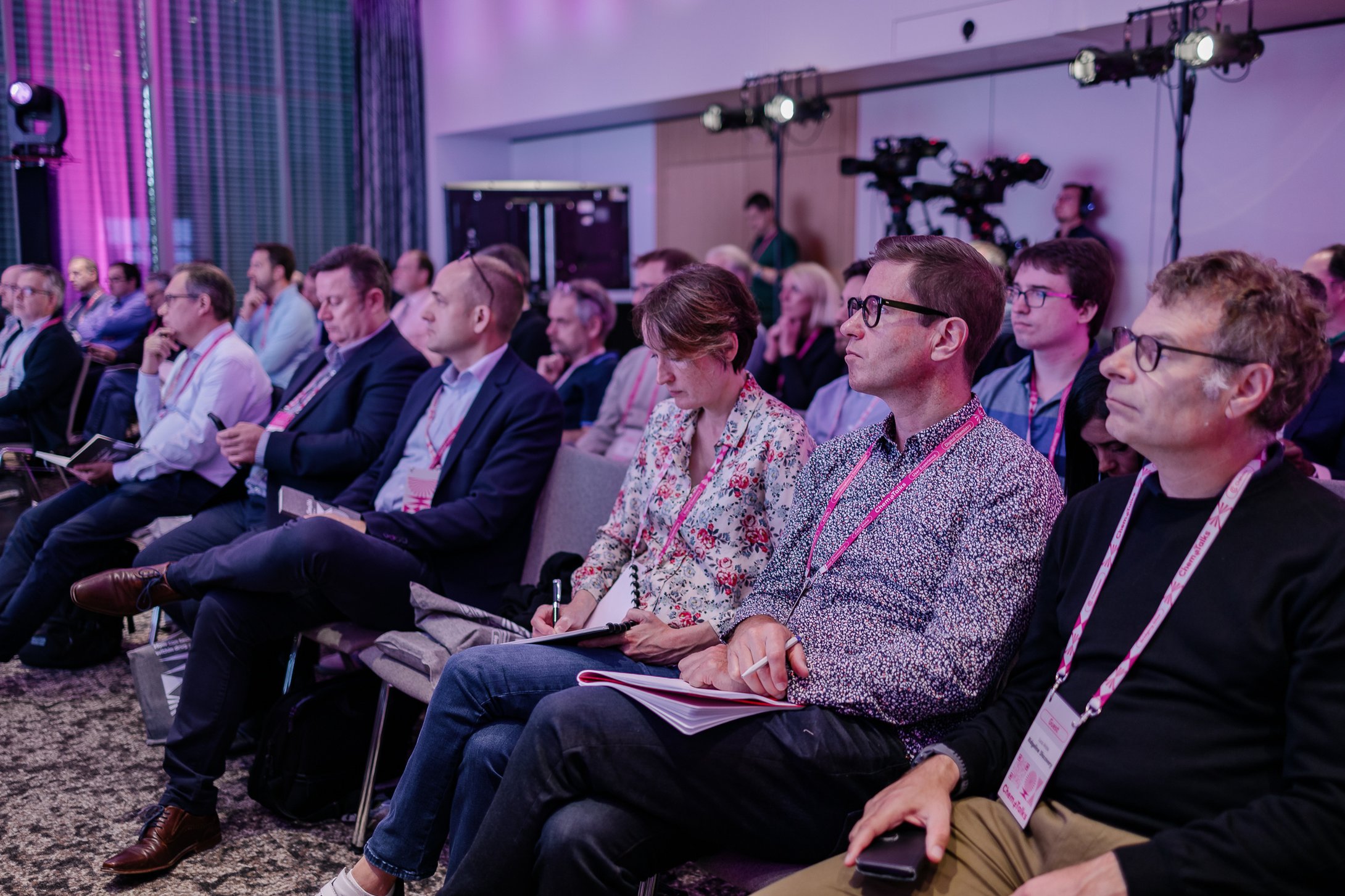
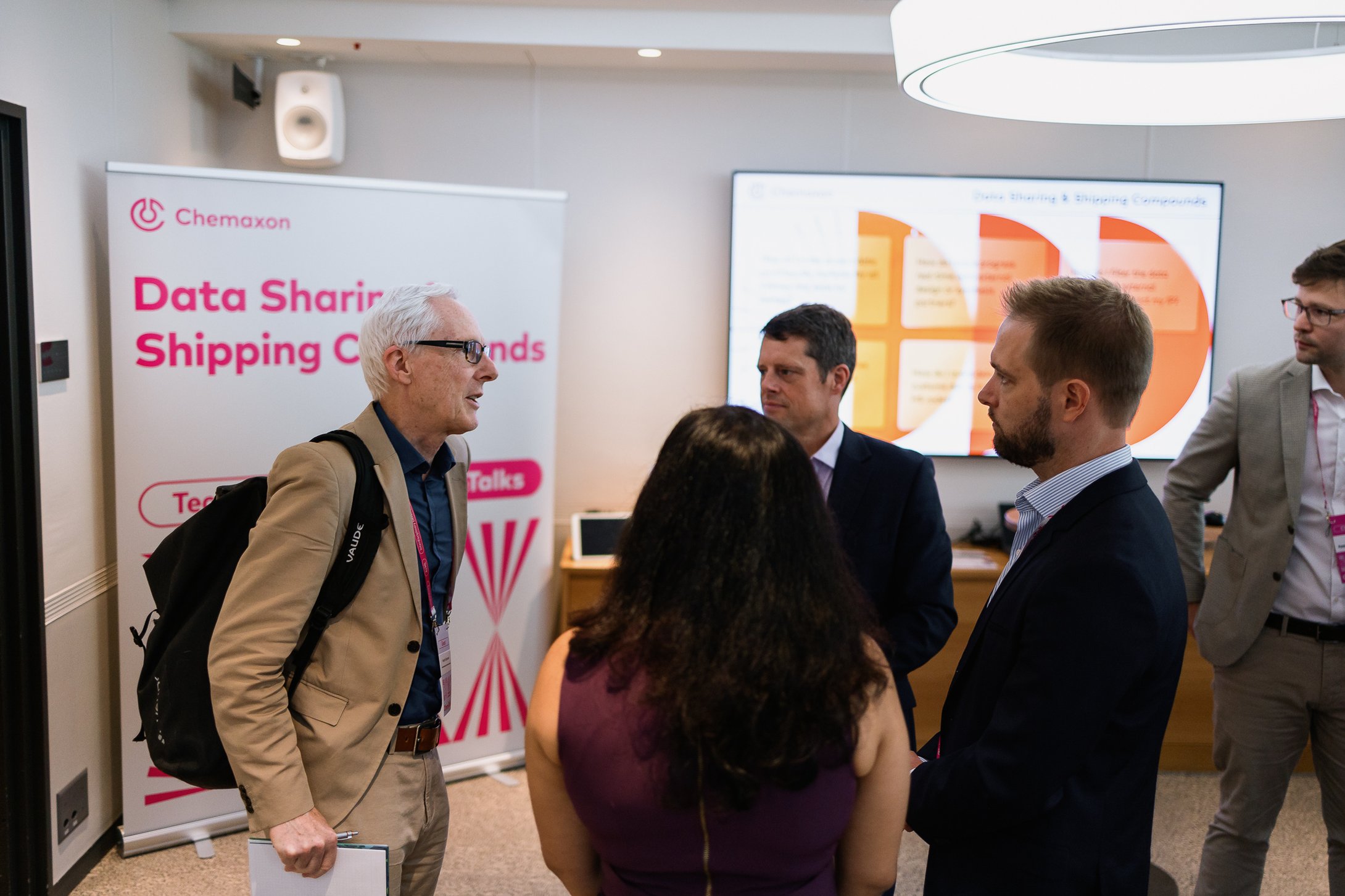
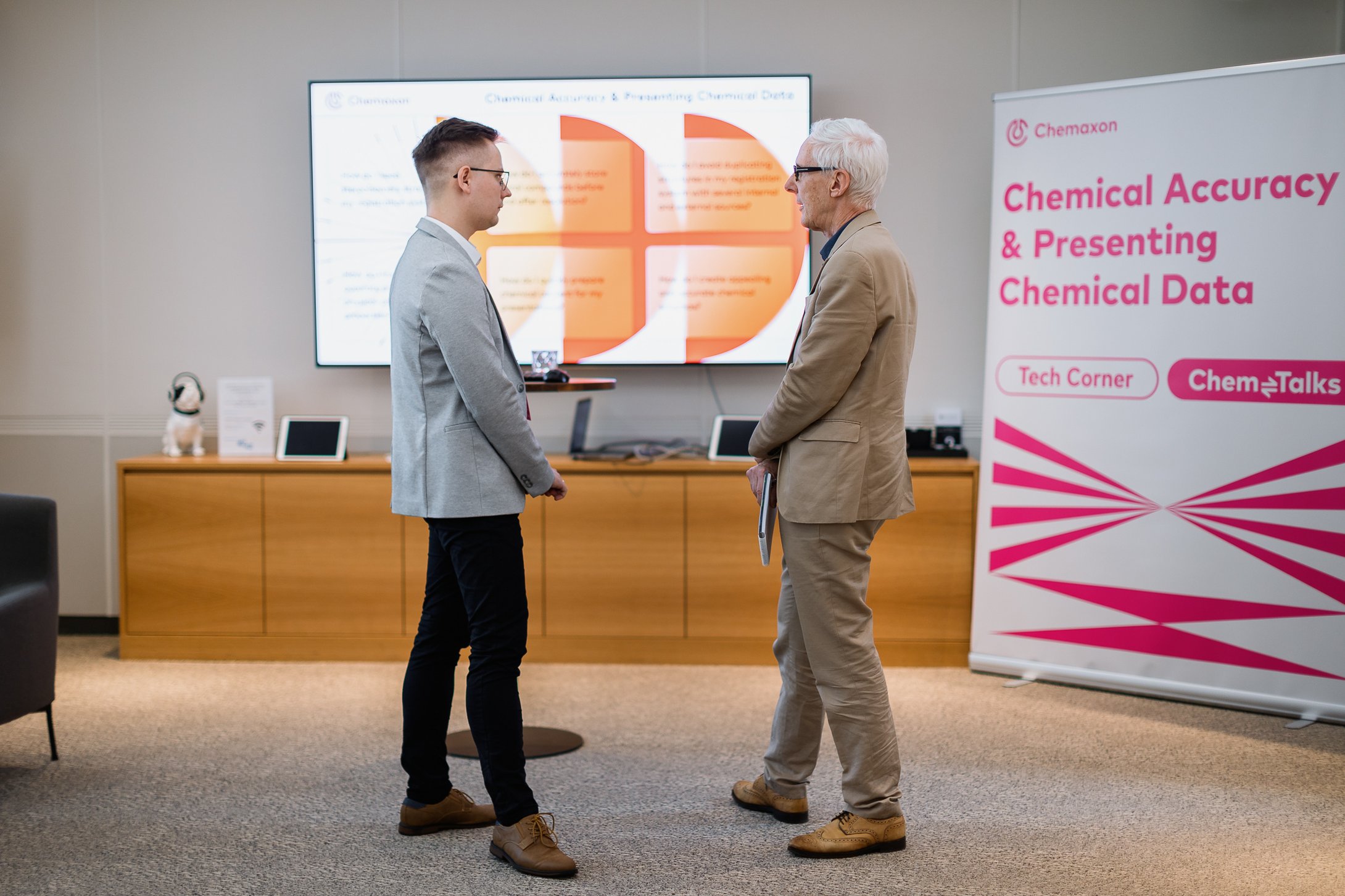
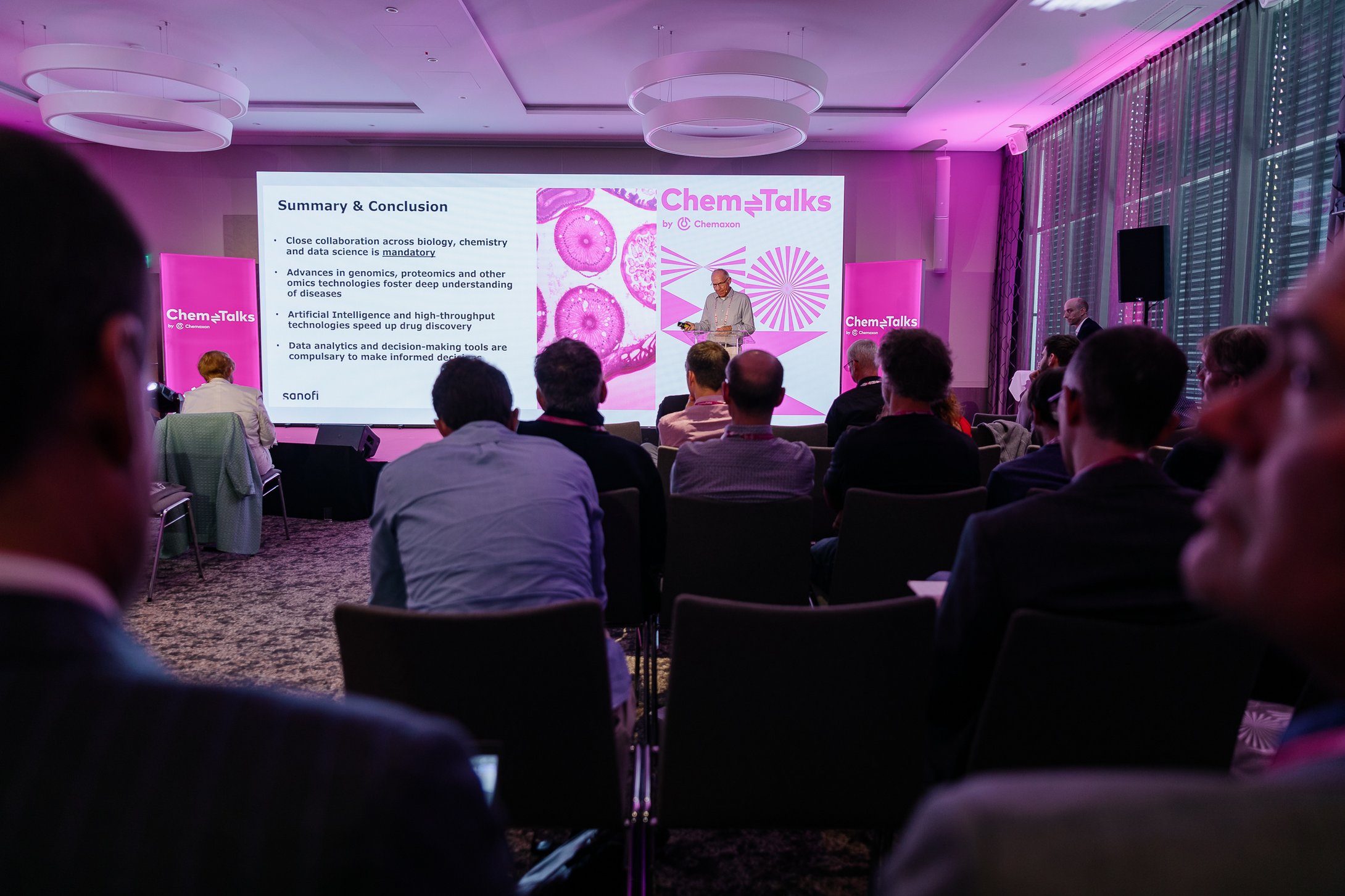
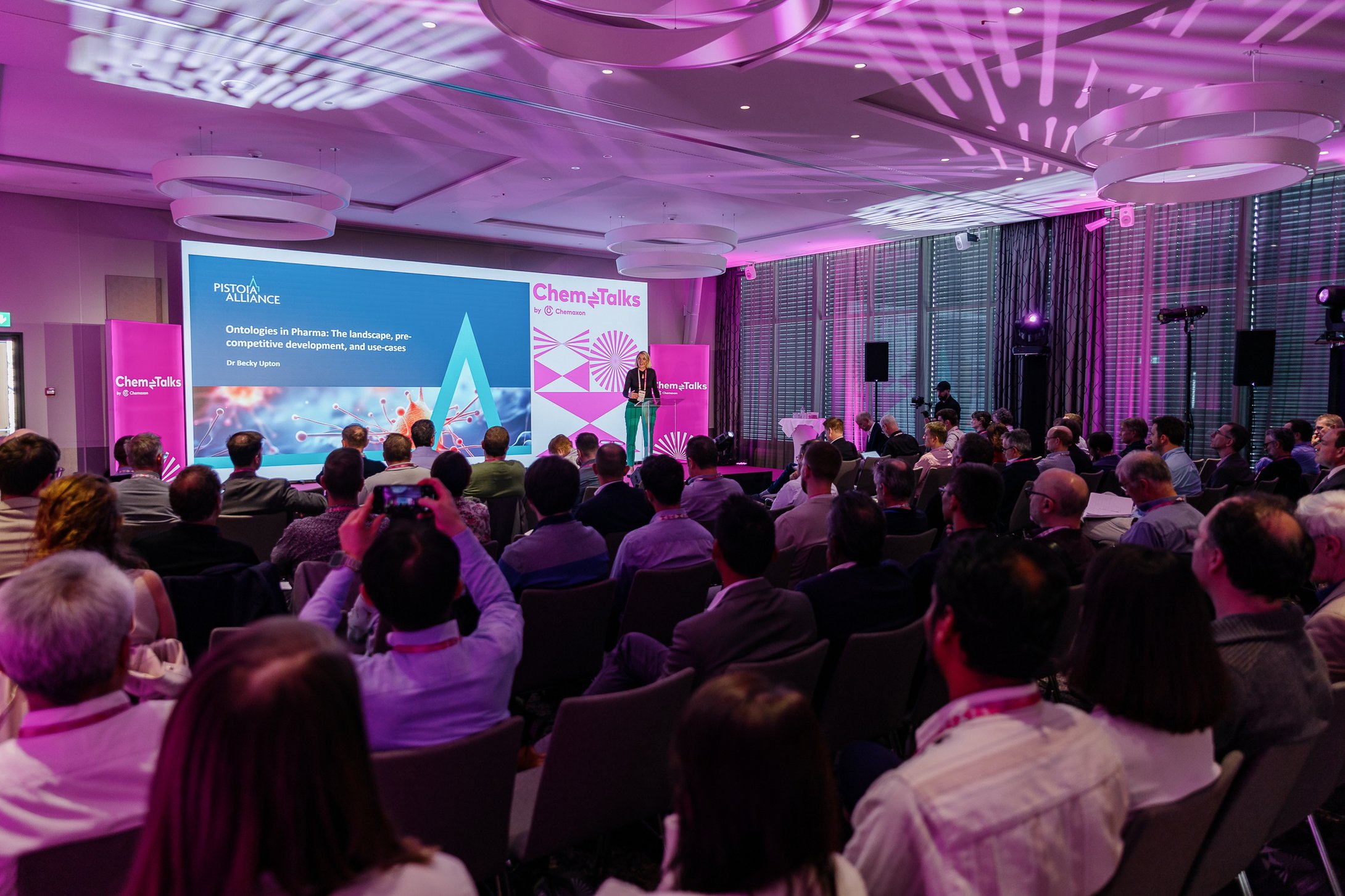
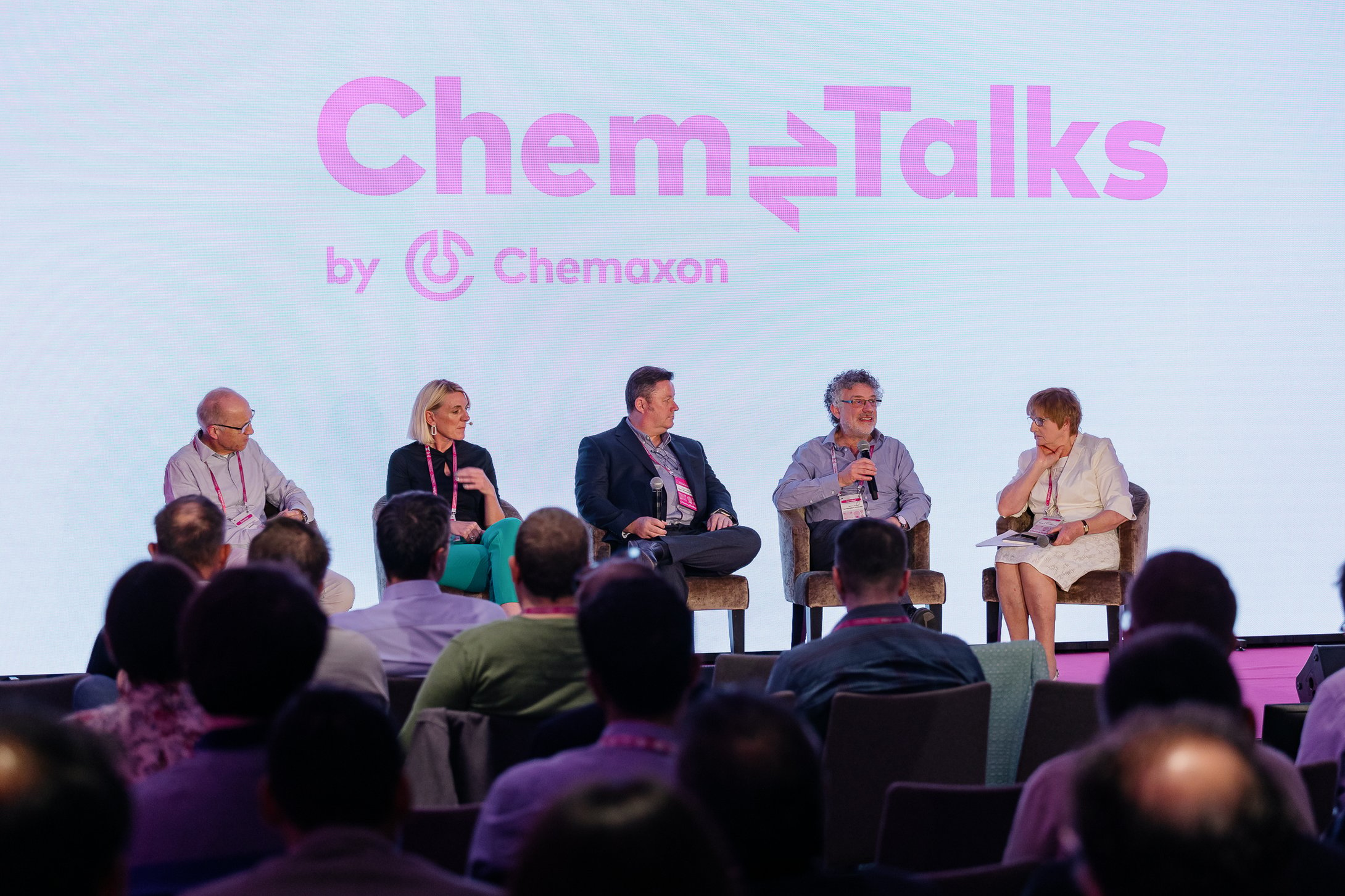
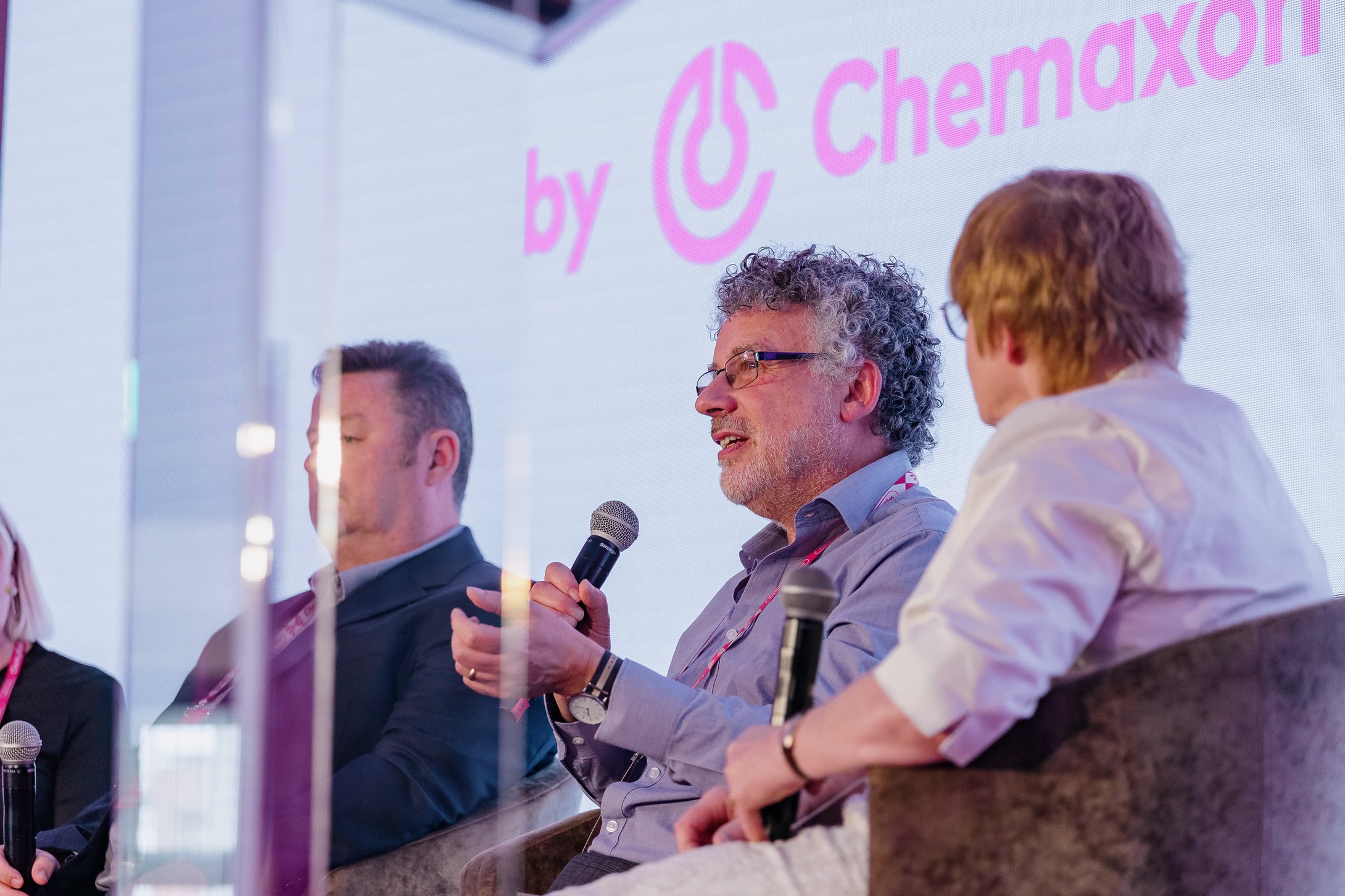
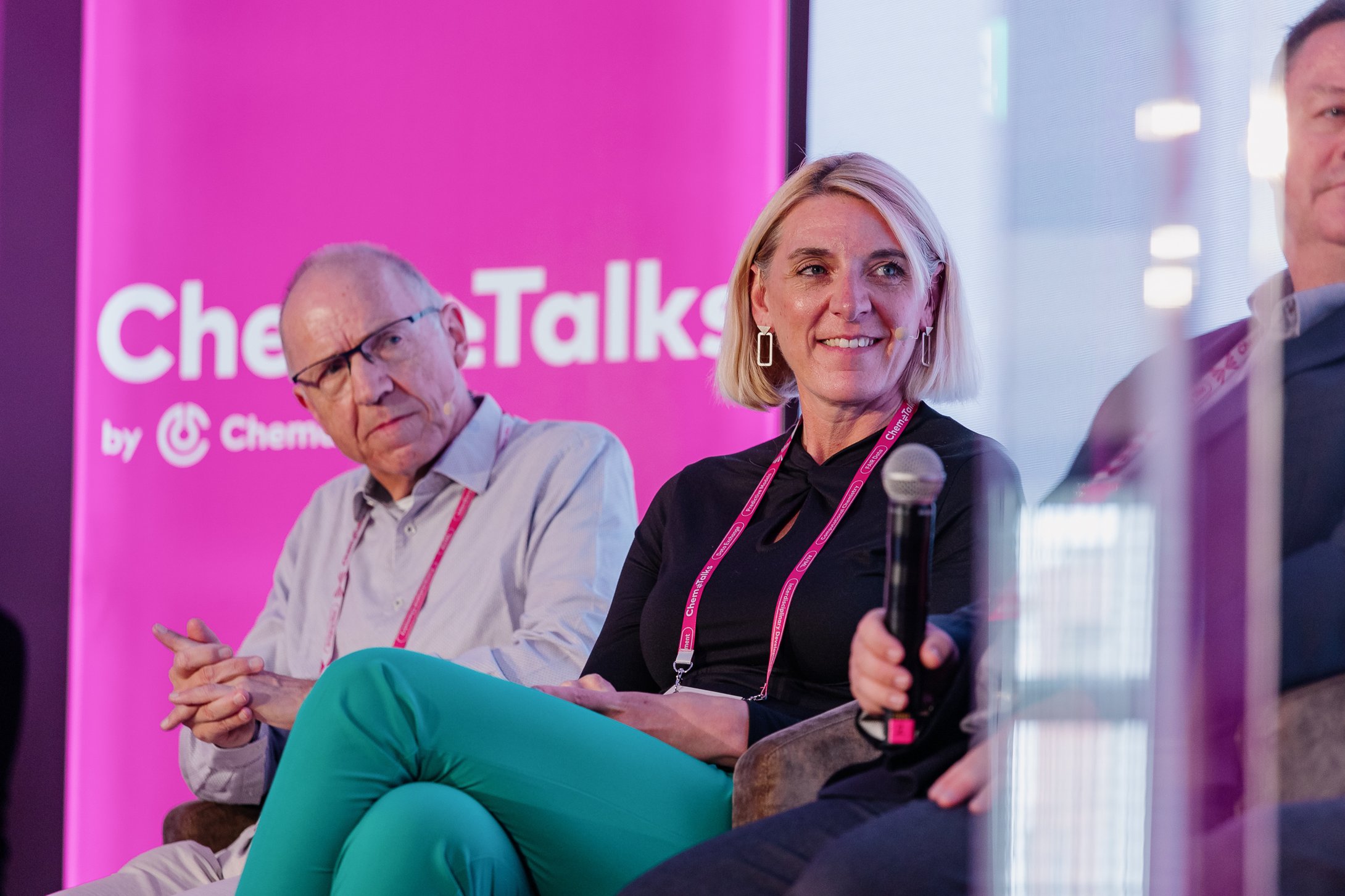
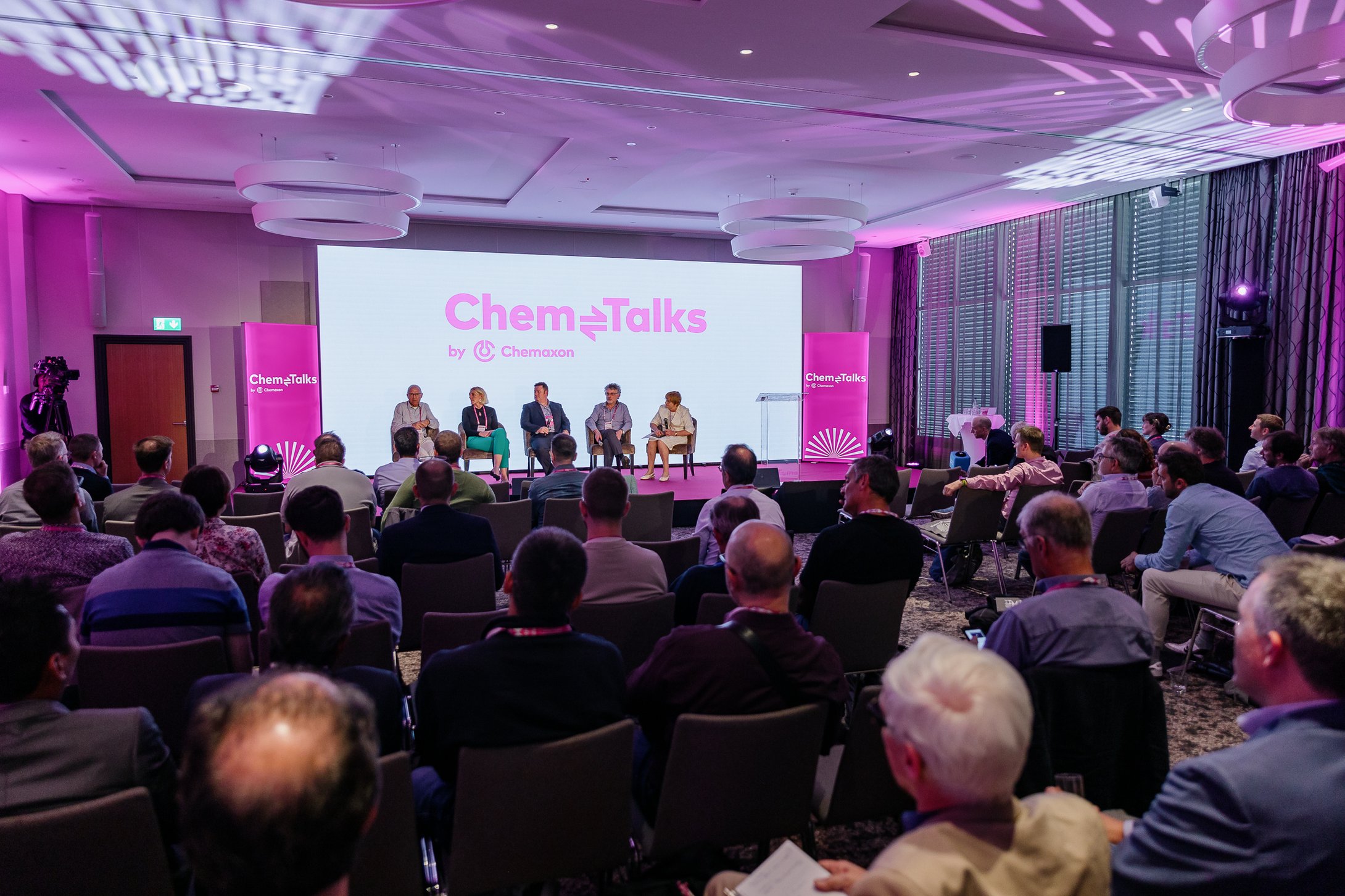
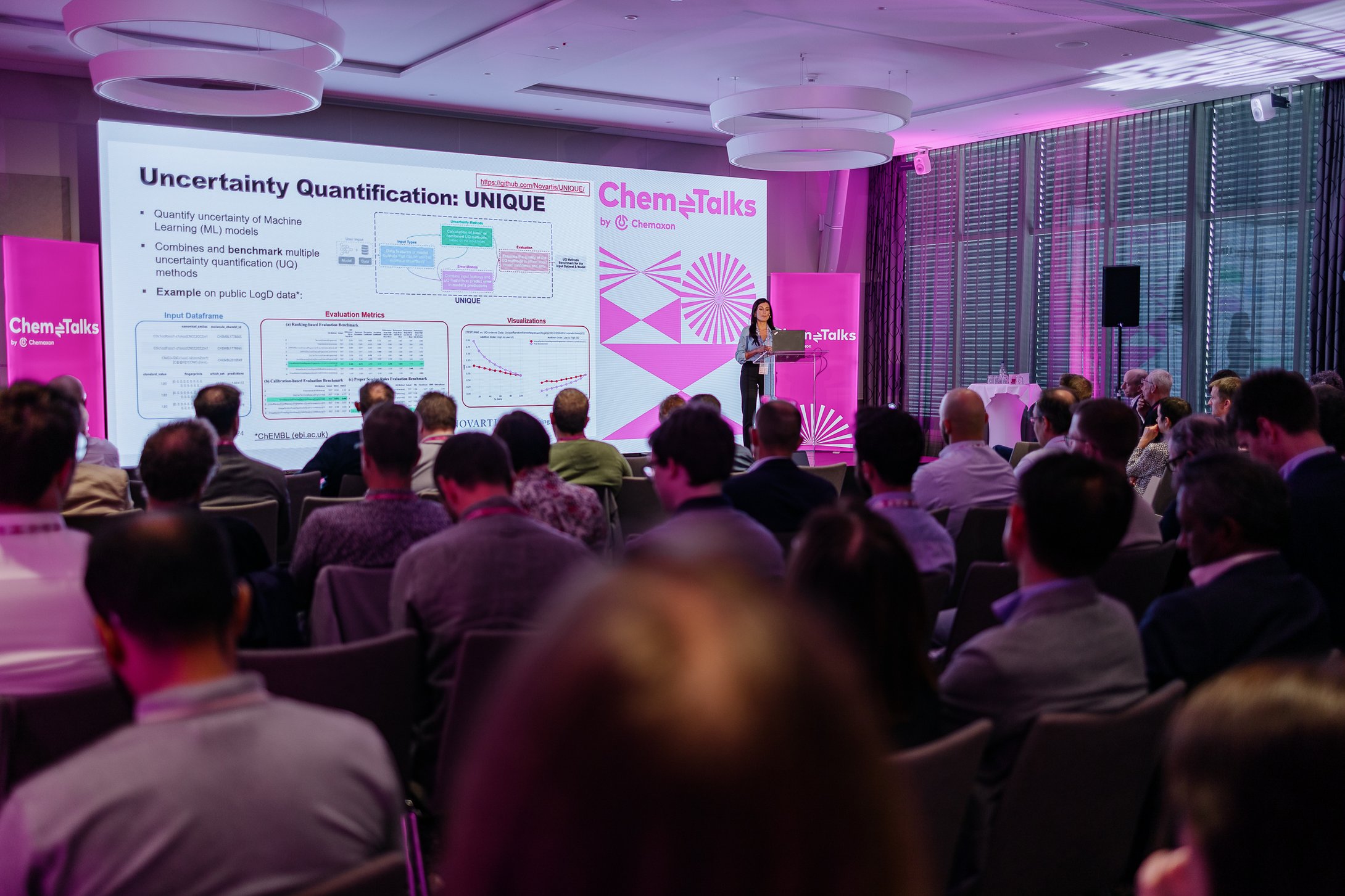
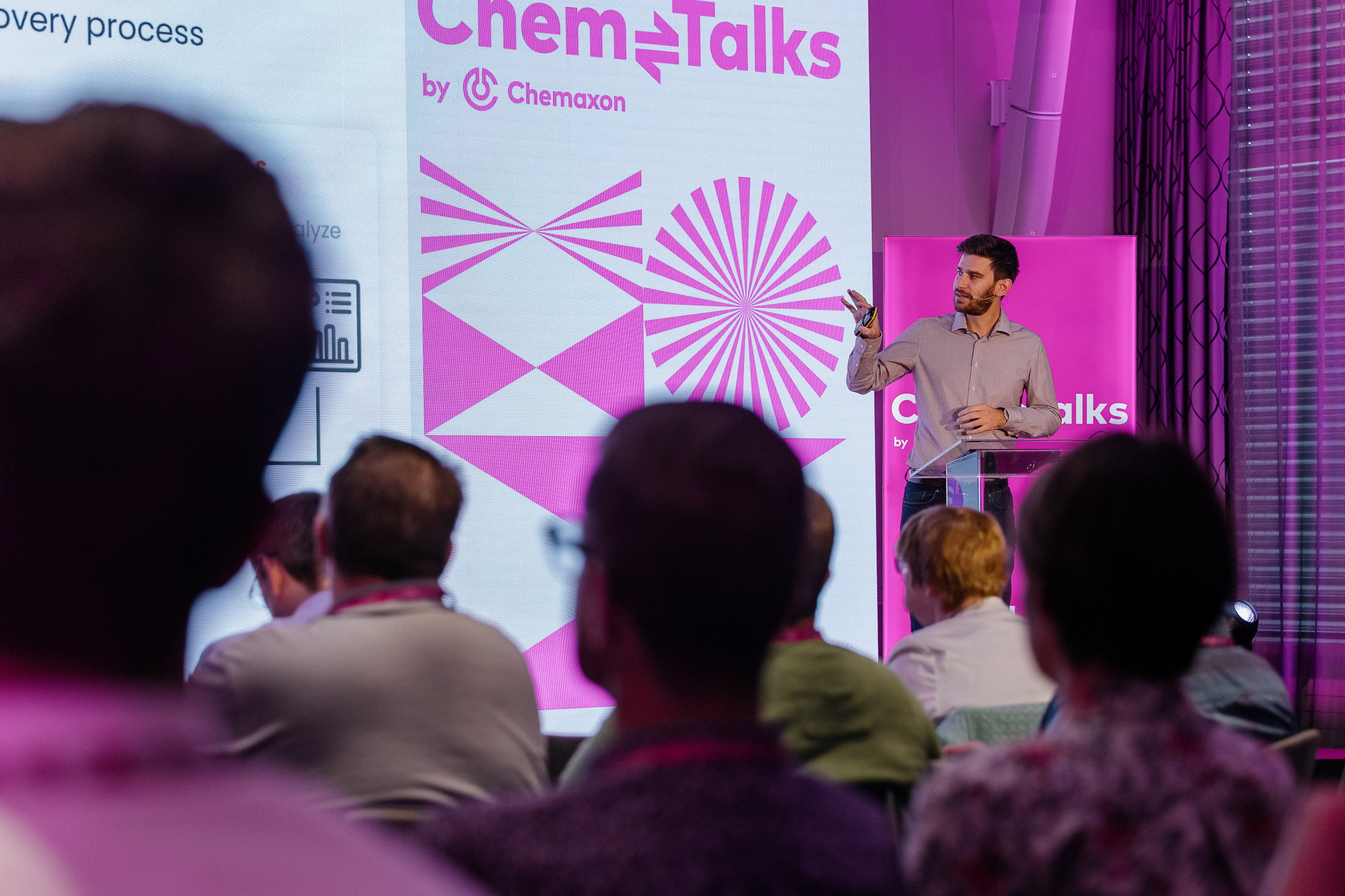
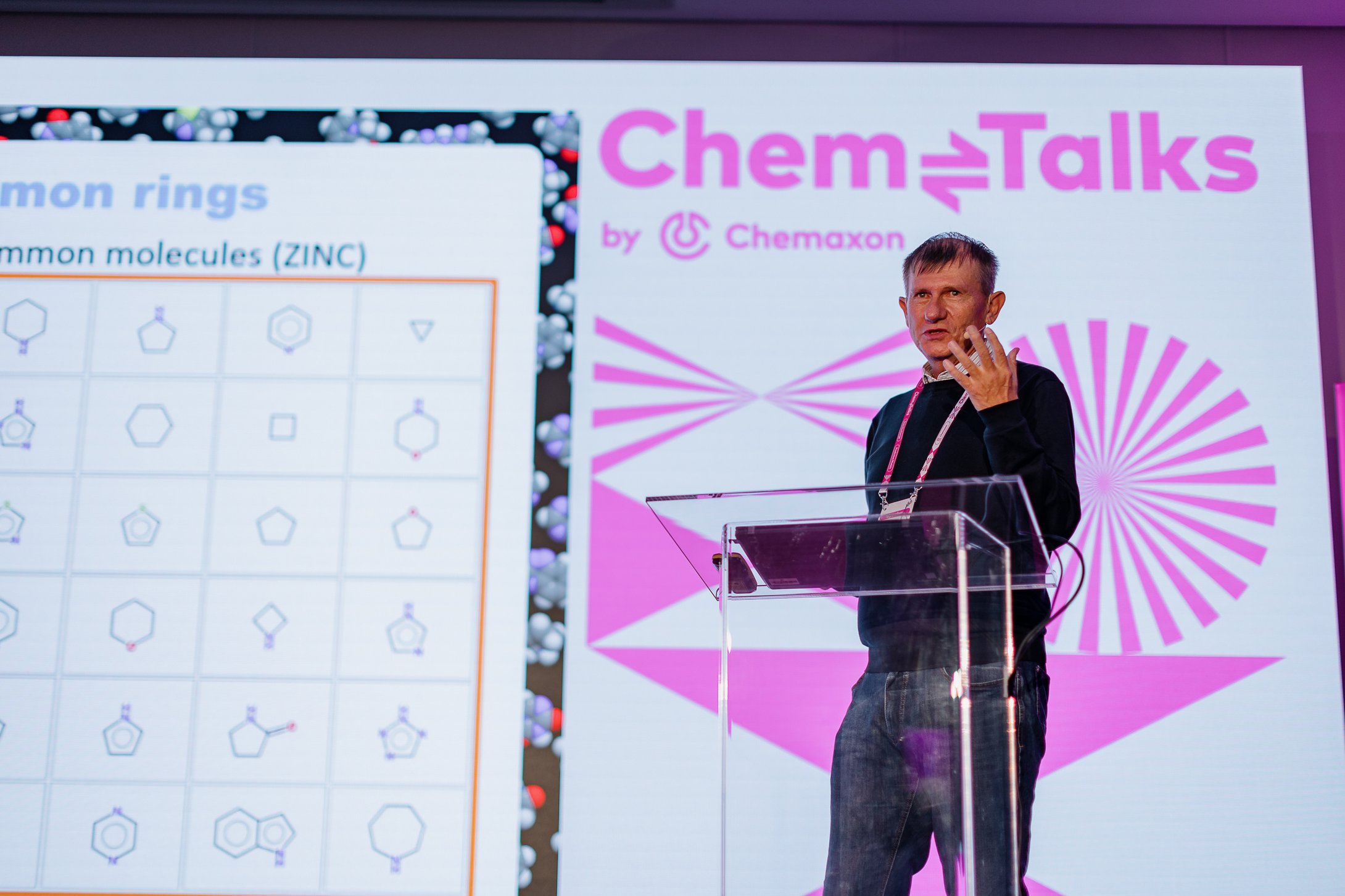
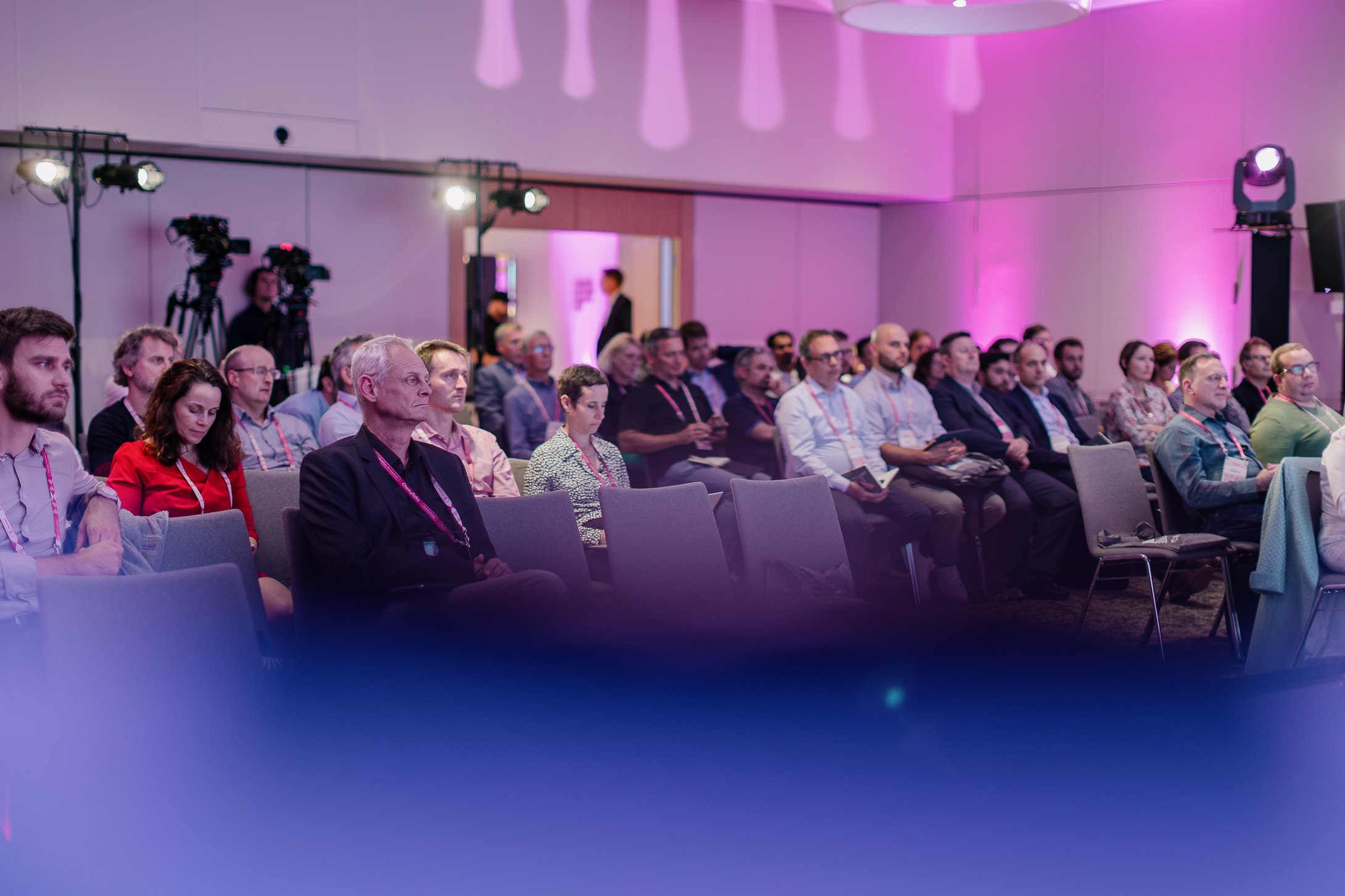
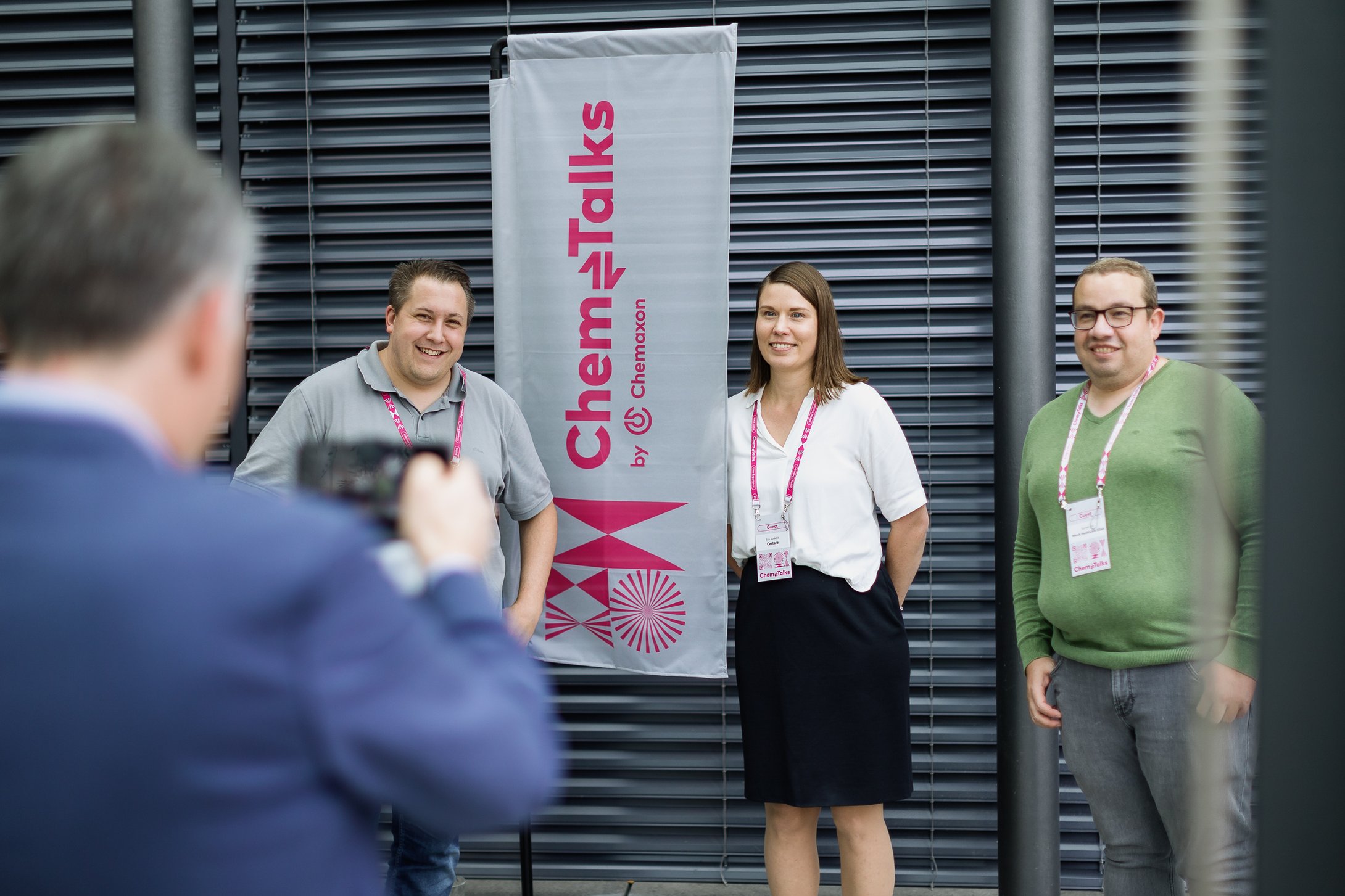
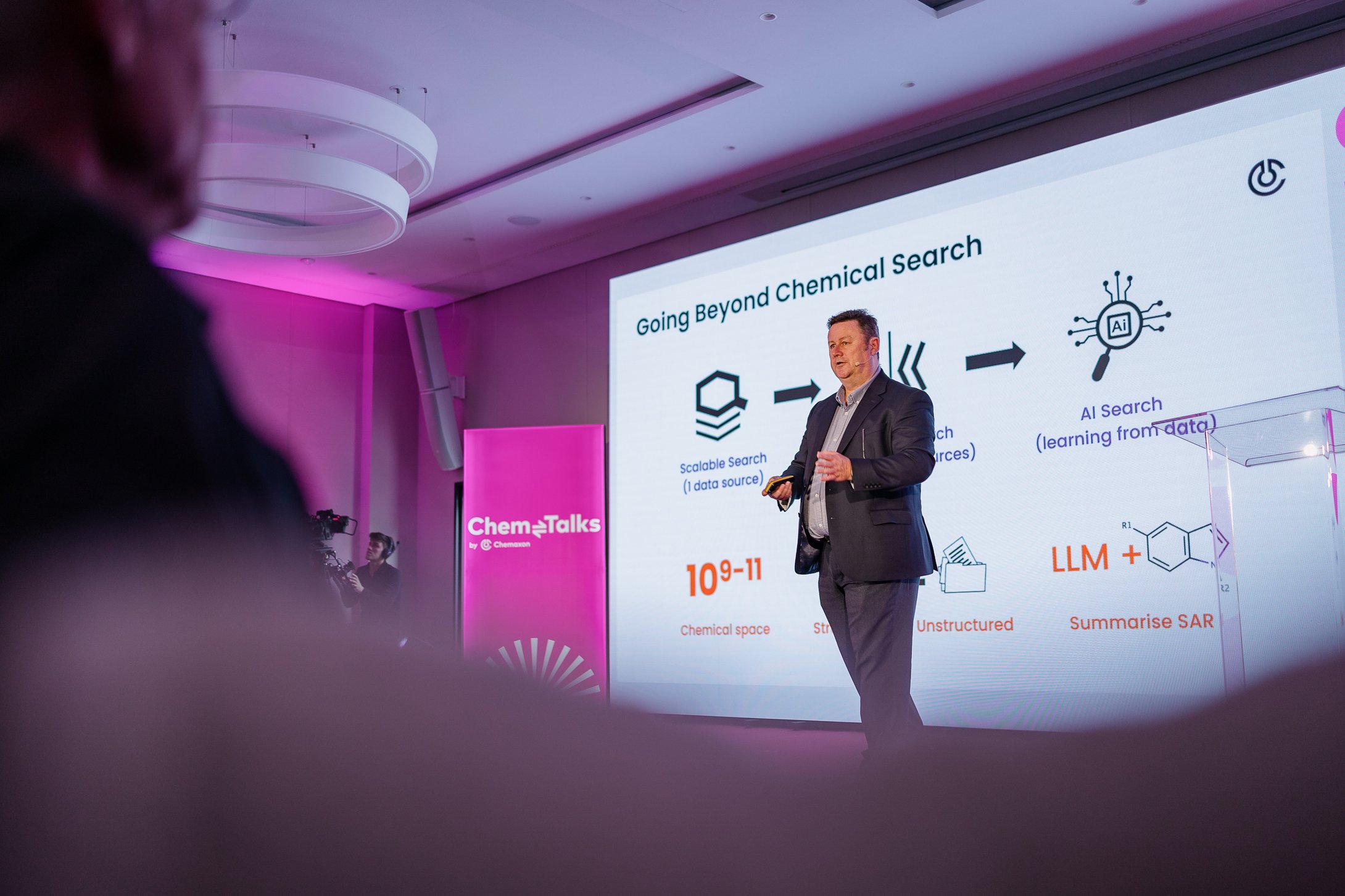
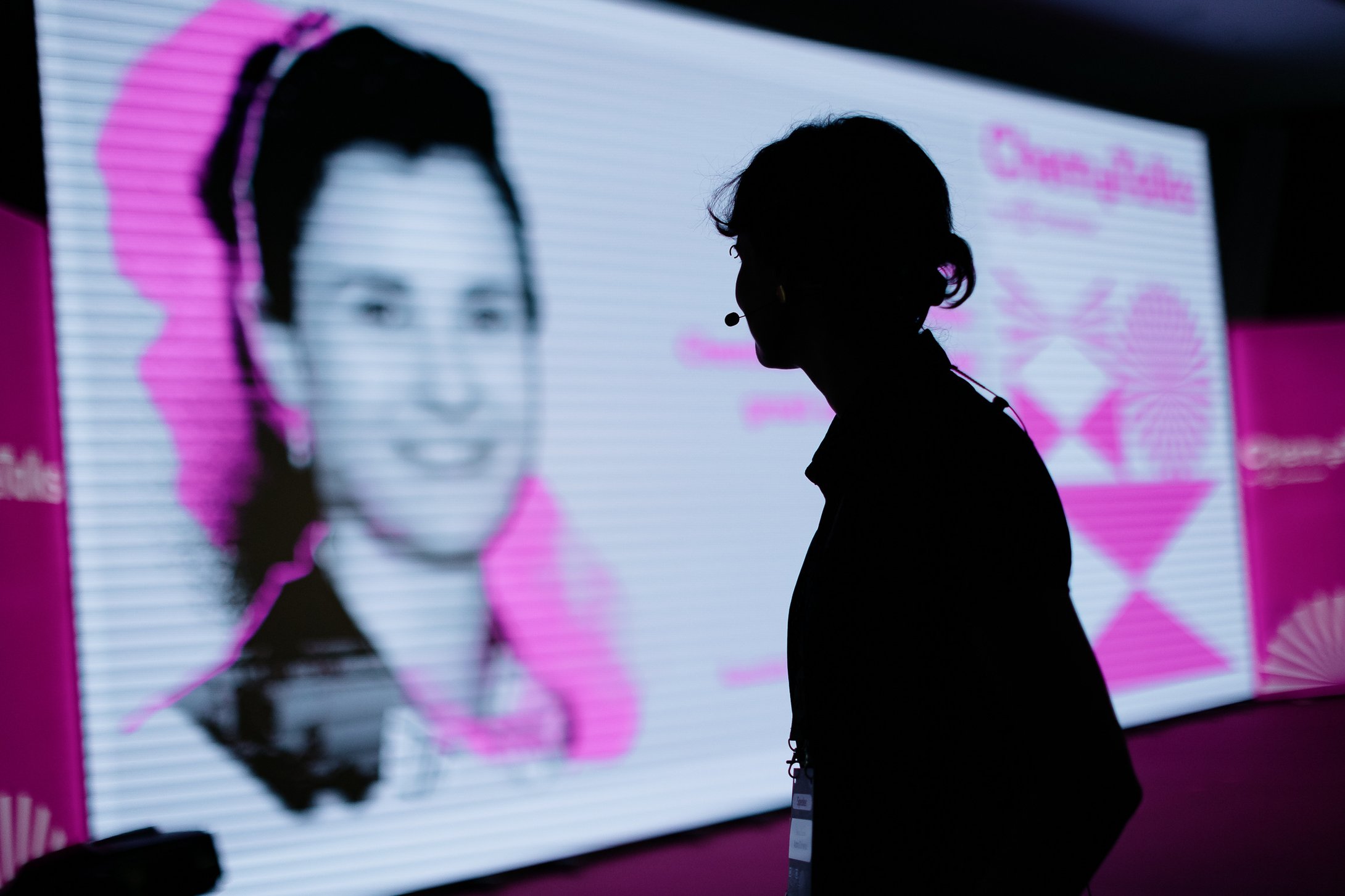
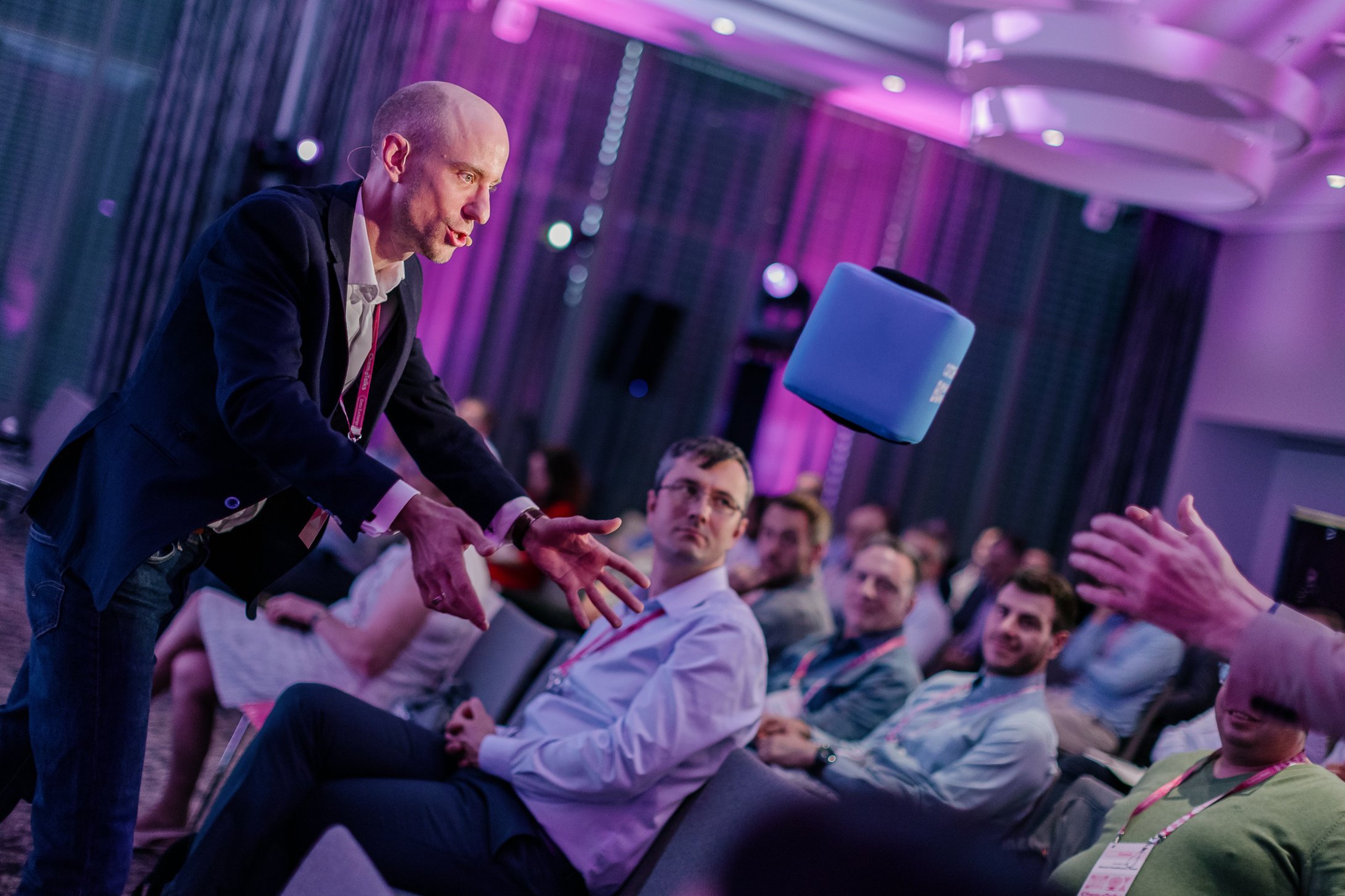
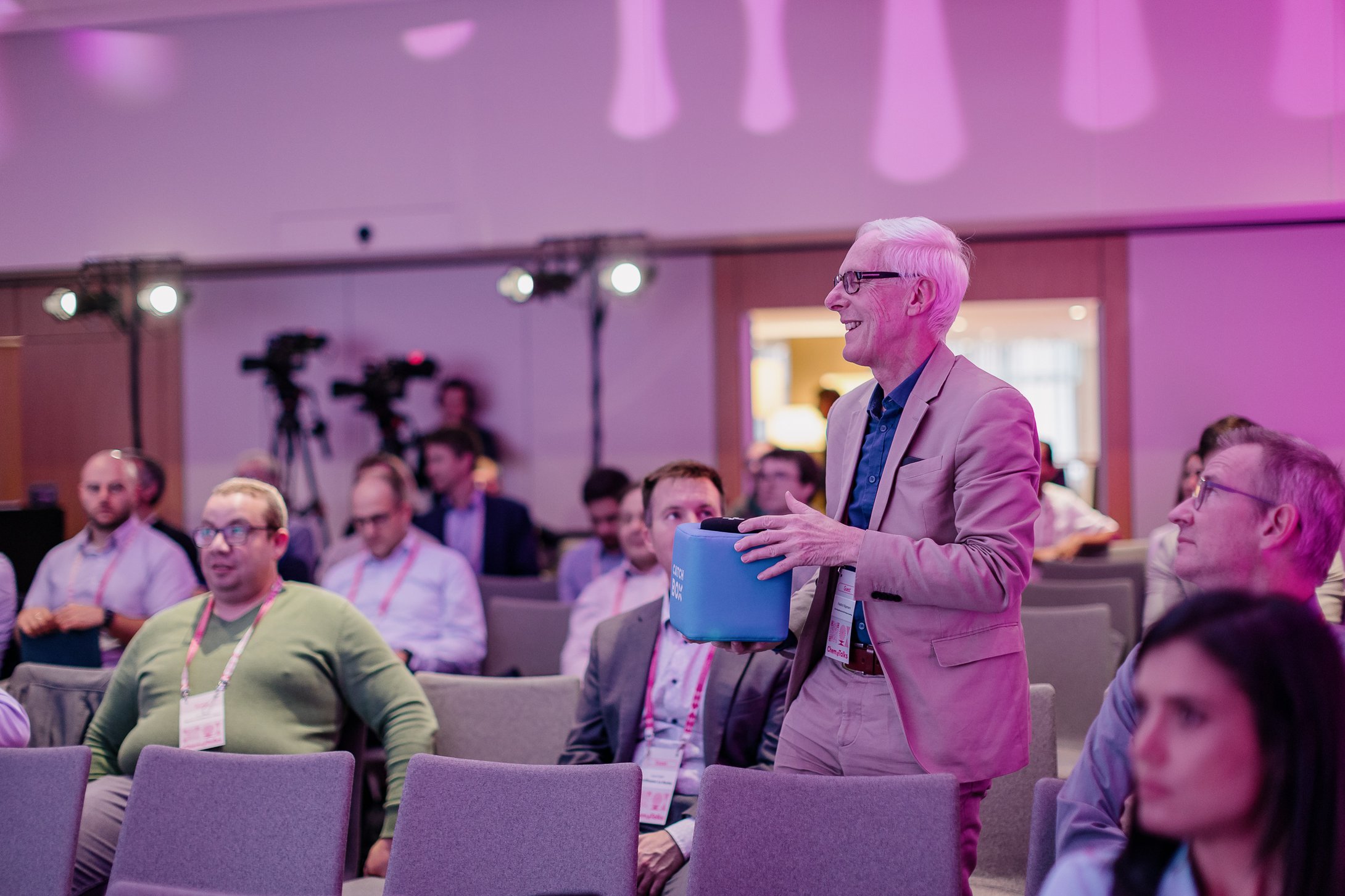
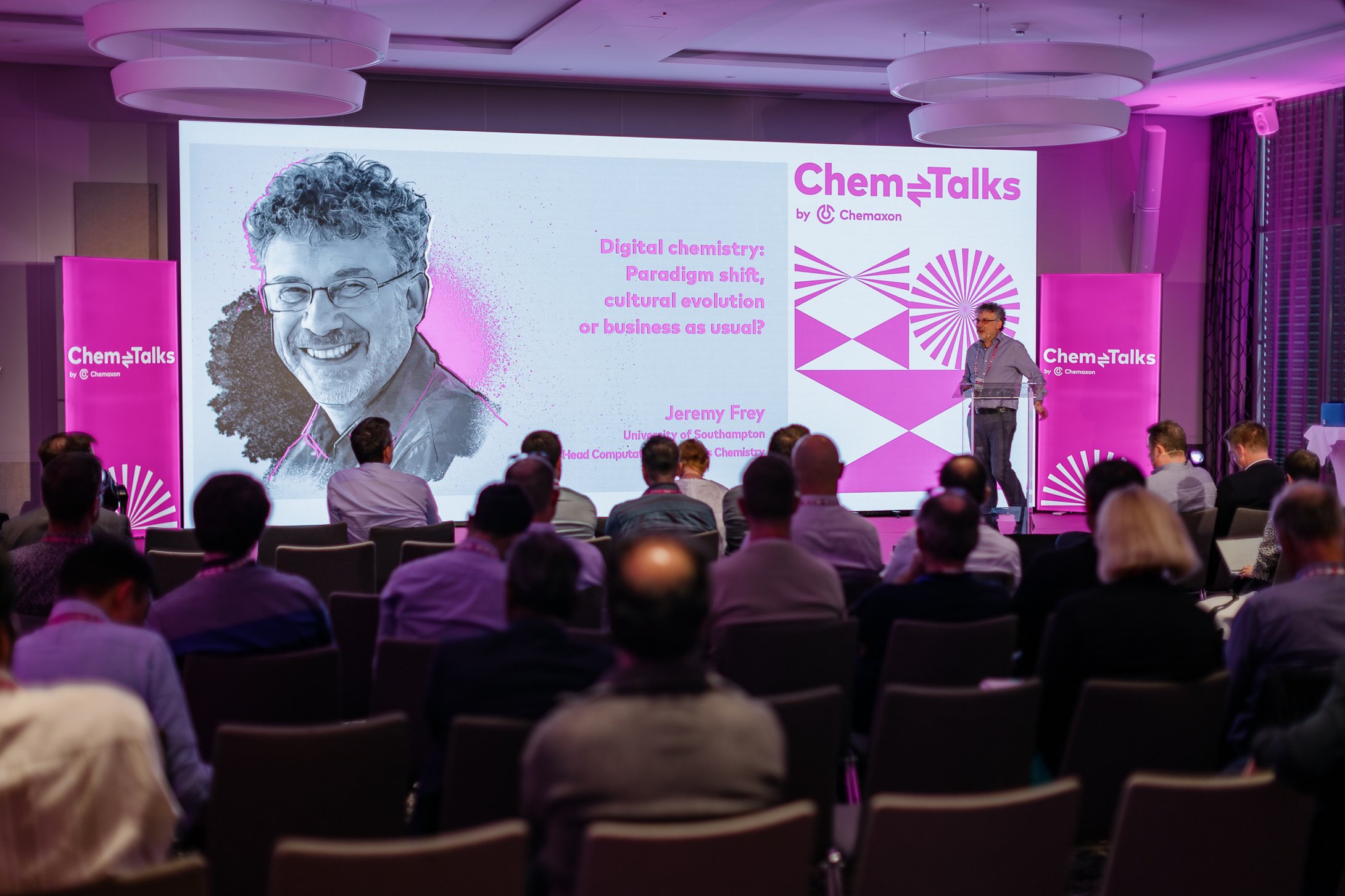
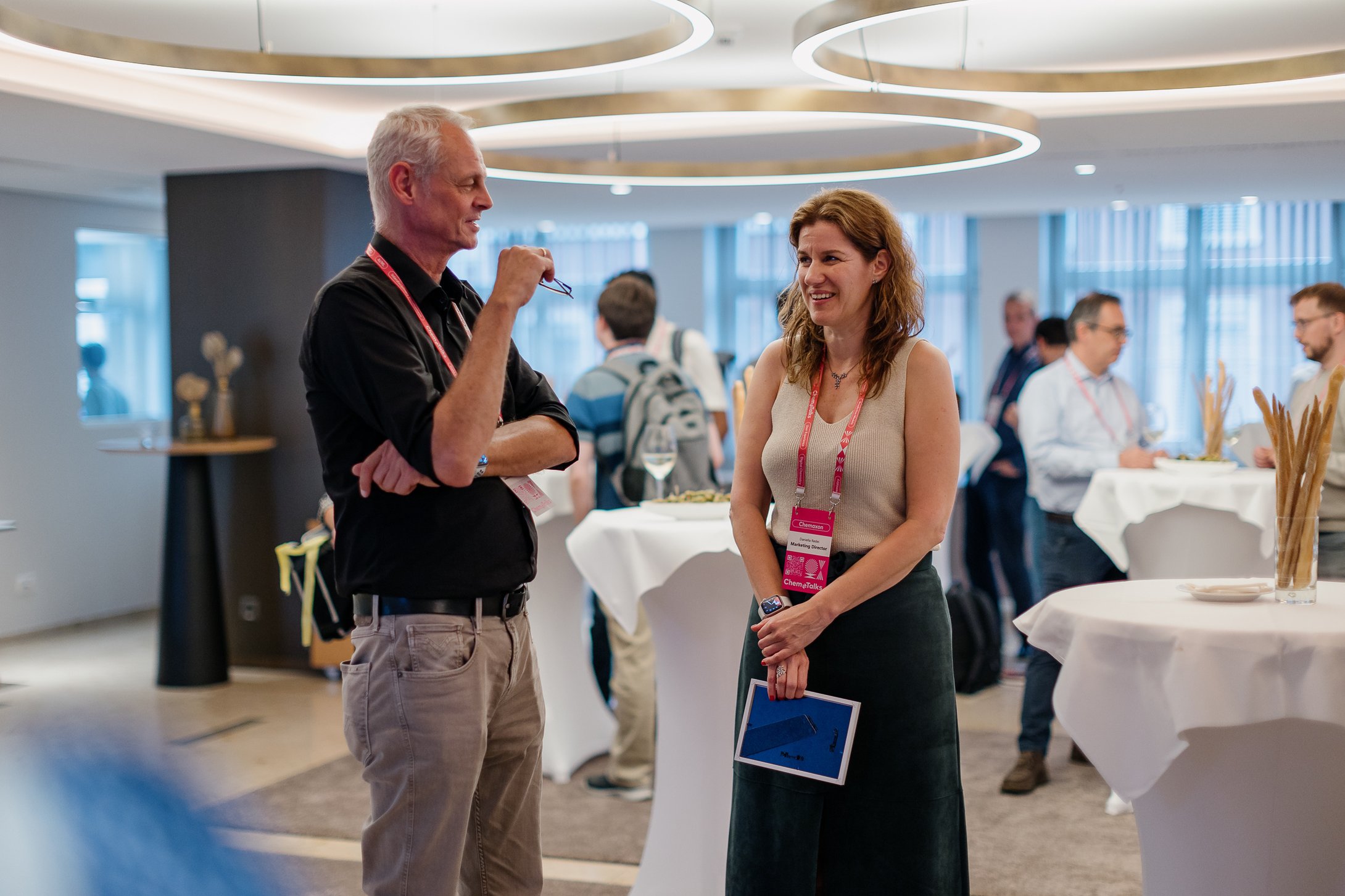
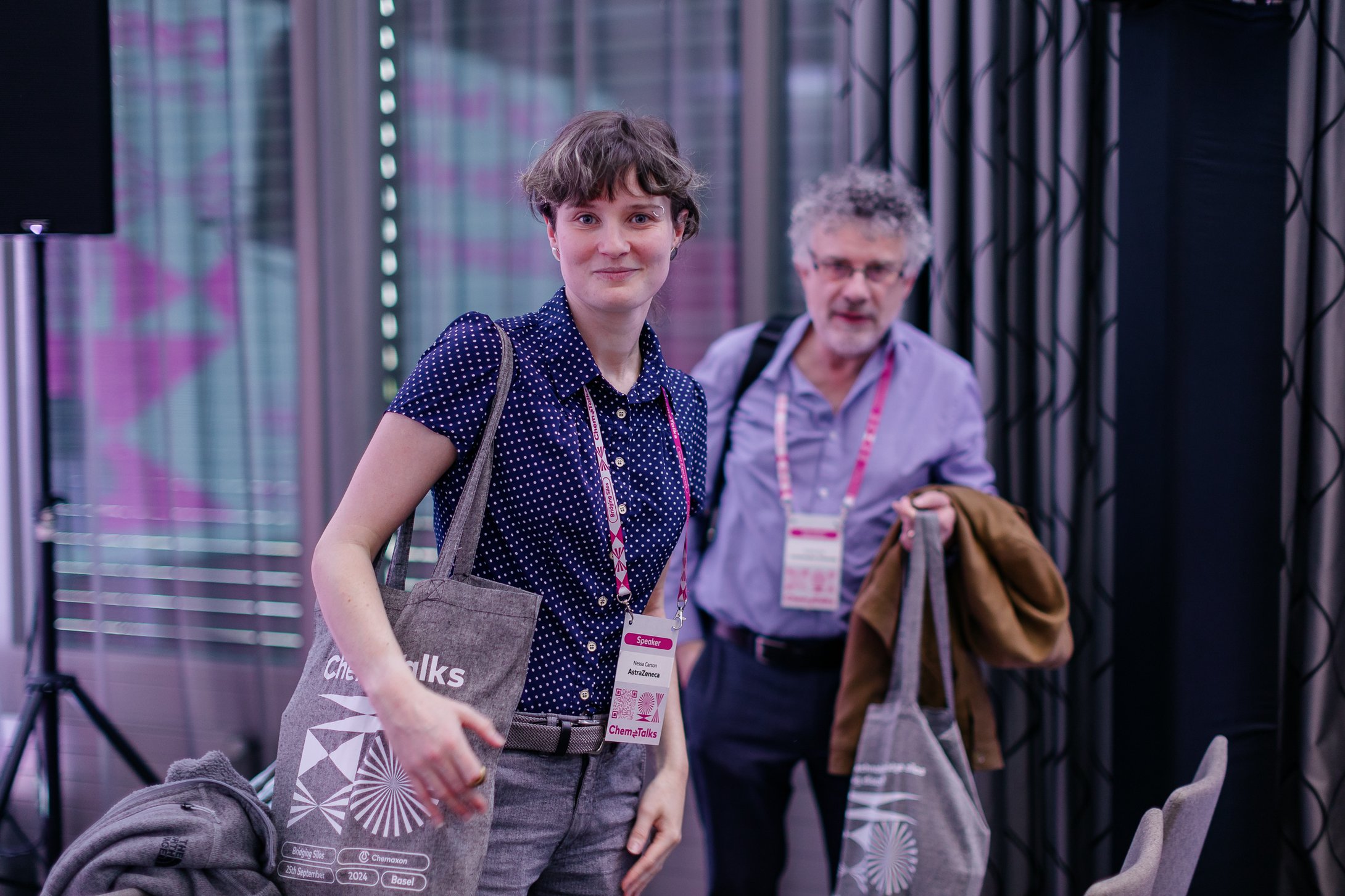
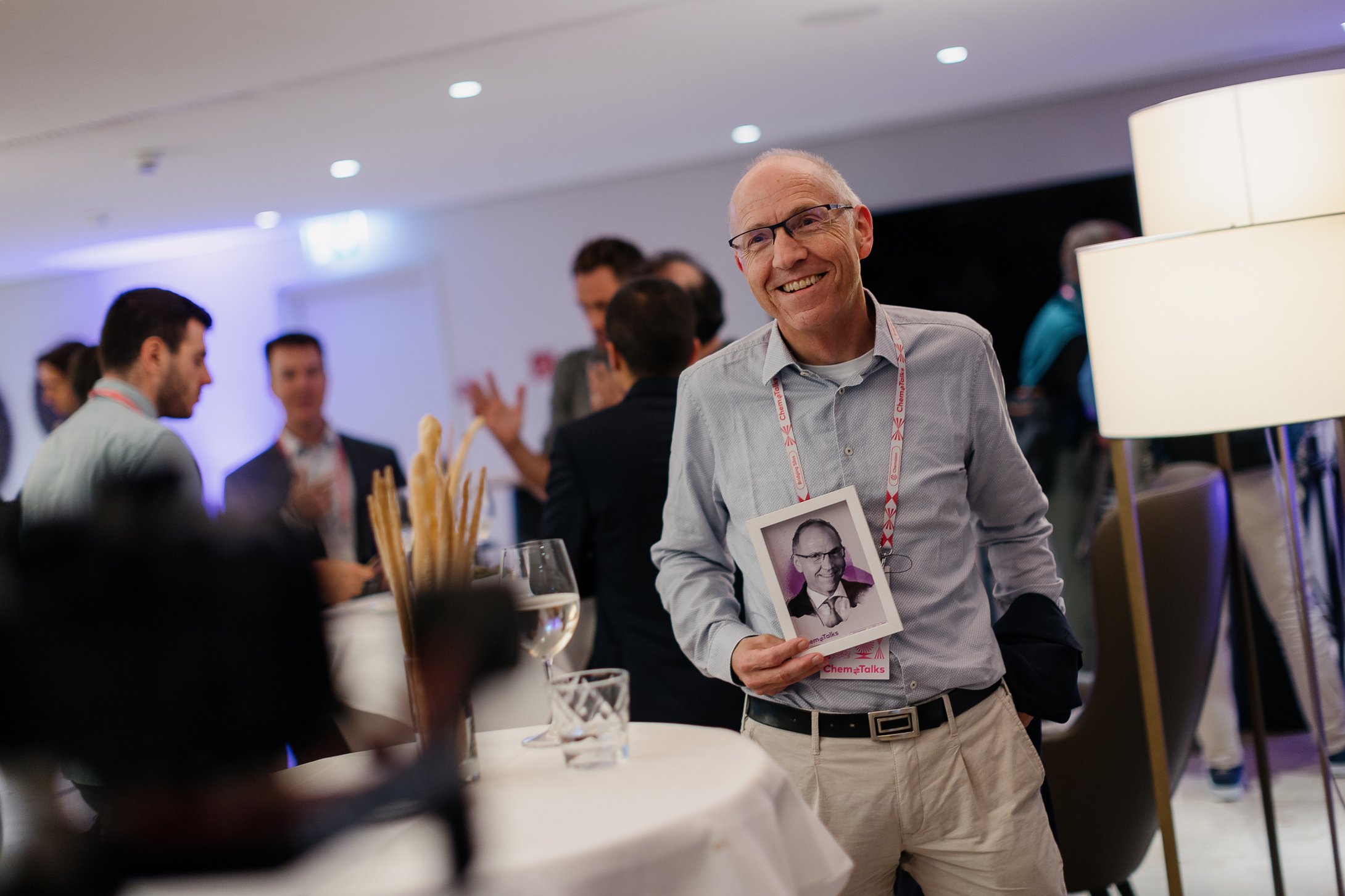
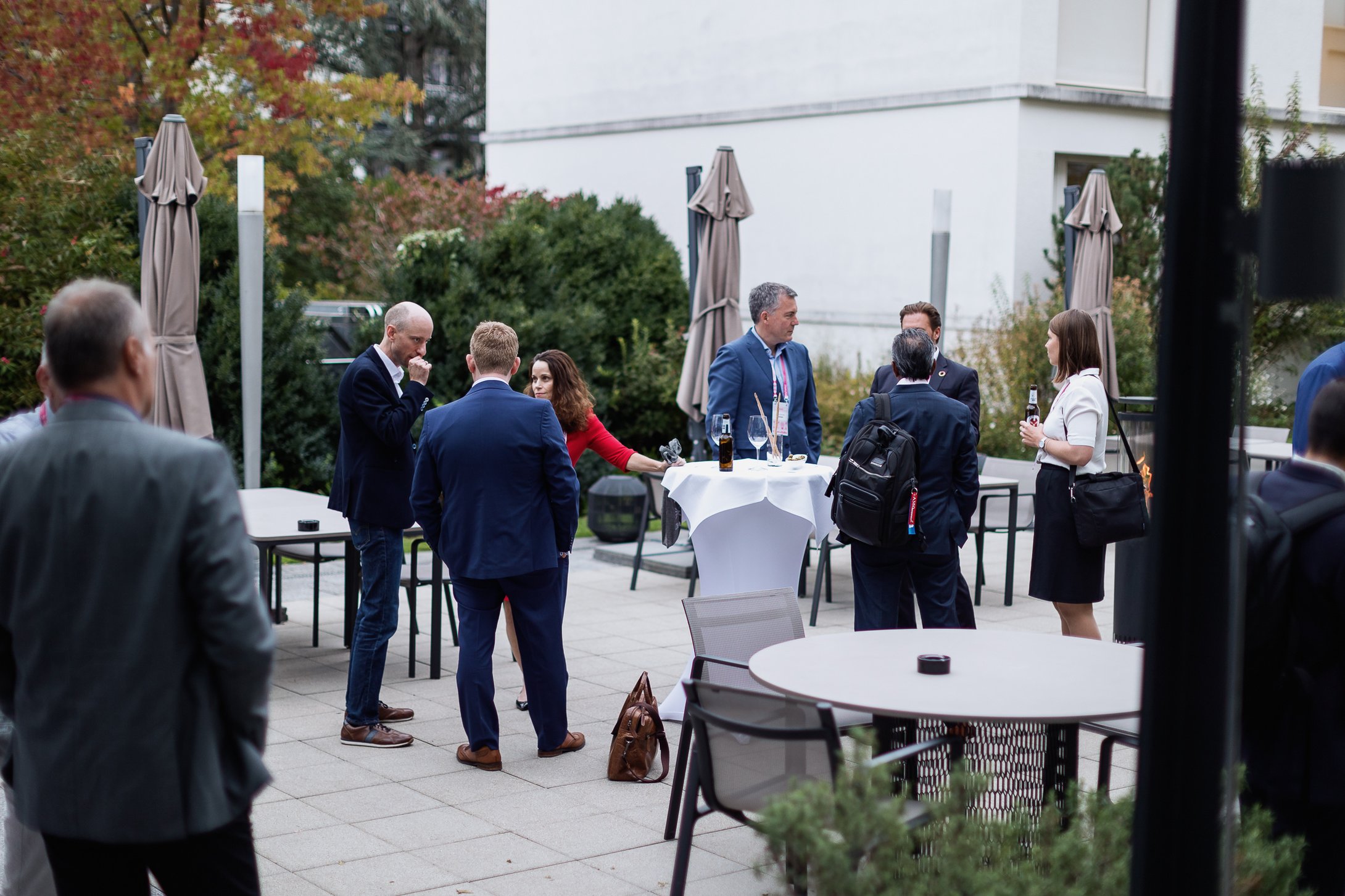
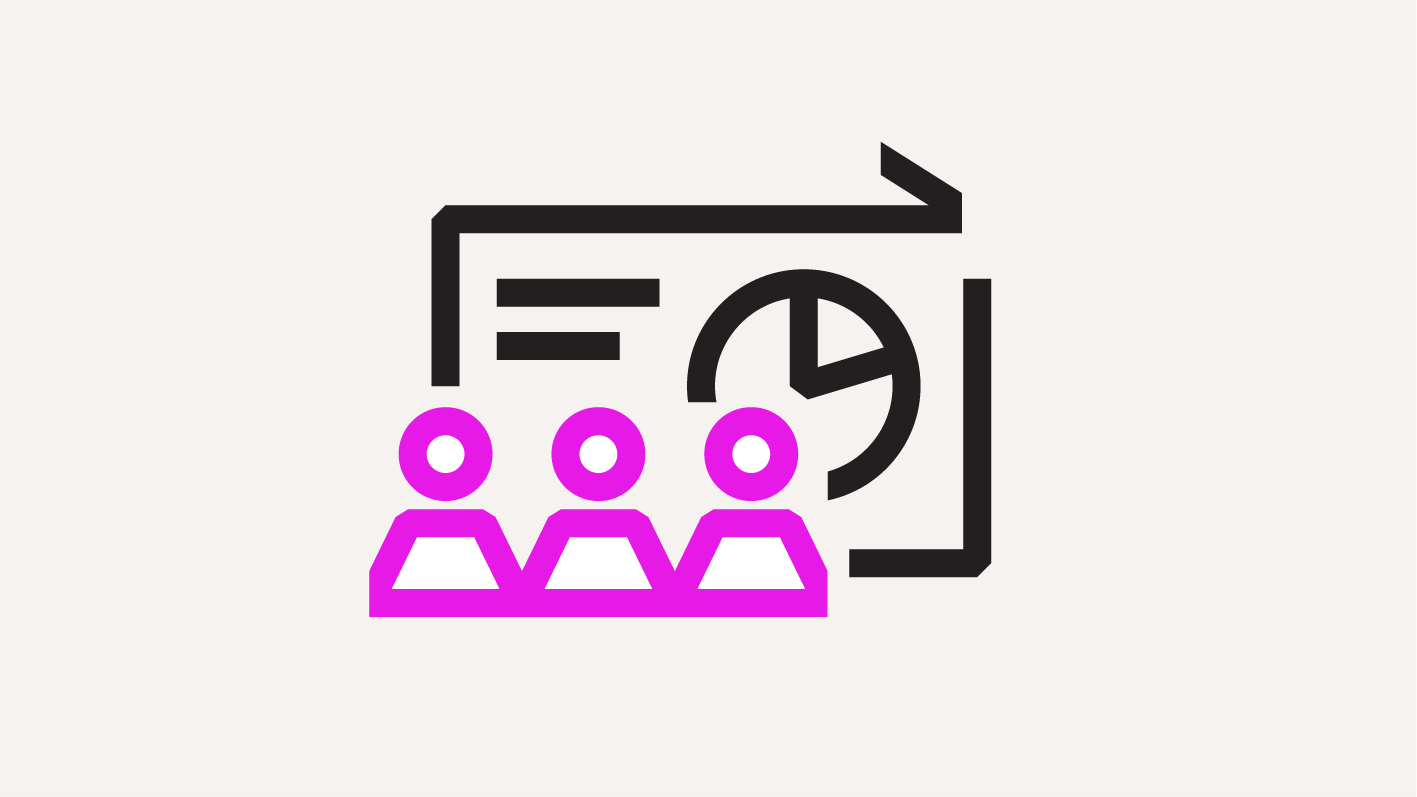
Learn from the best
Hear from top experts sharing their stories on both their successes and current challenges.
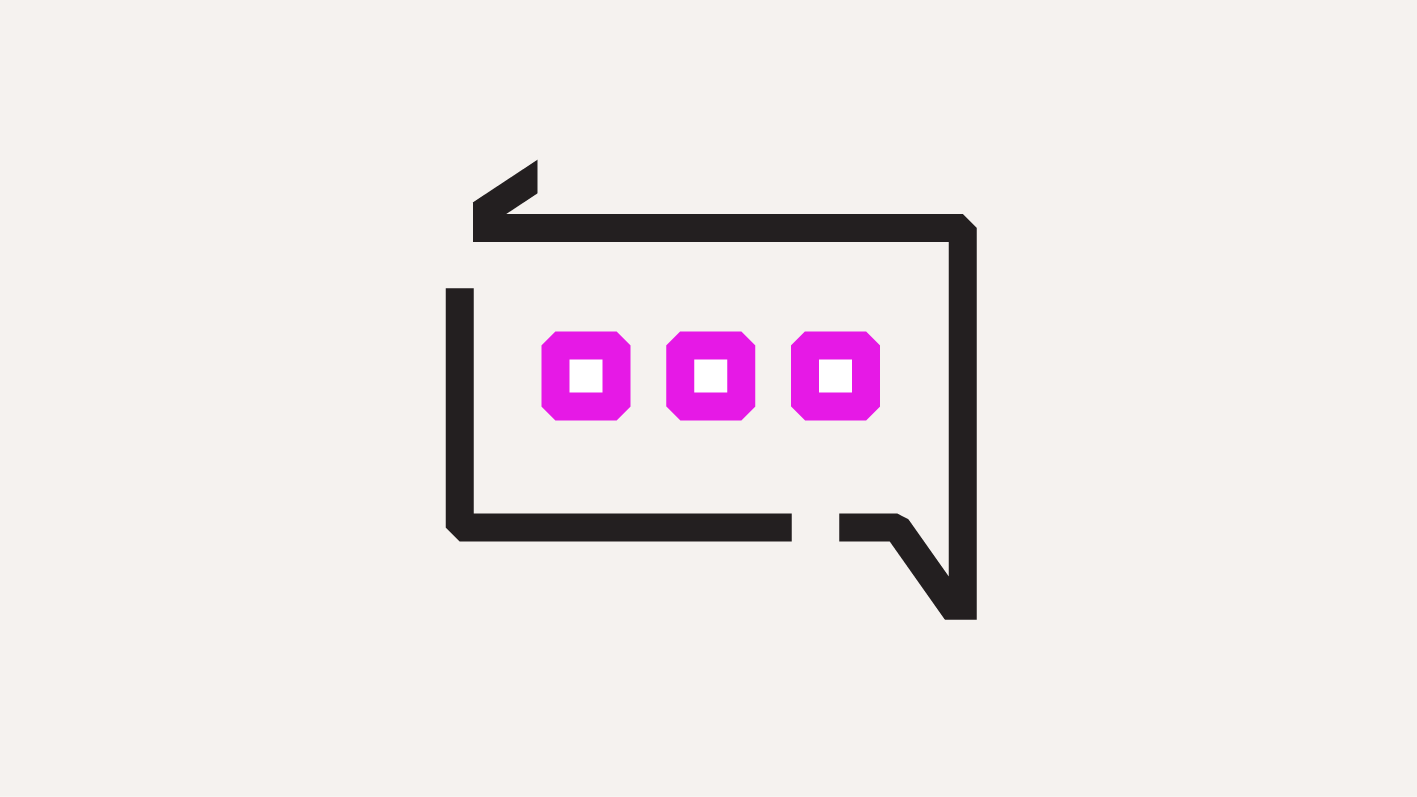
Join the conversation
Ask your questions and throw in your ideas. The goal is to learn from each other and grow together.
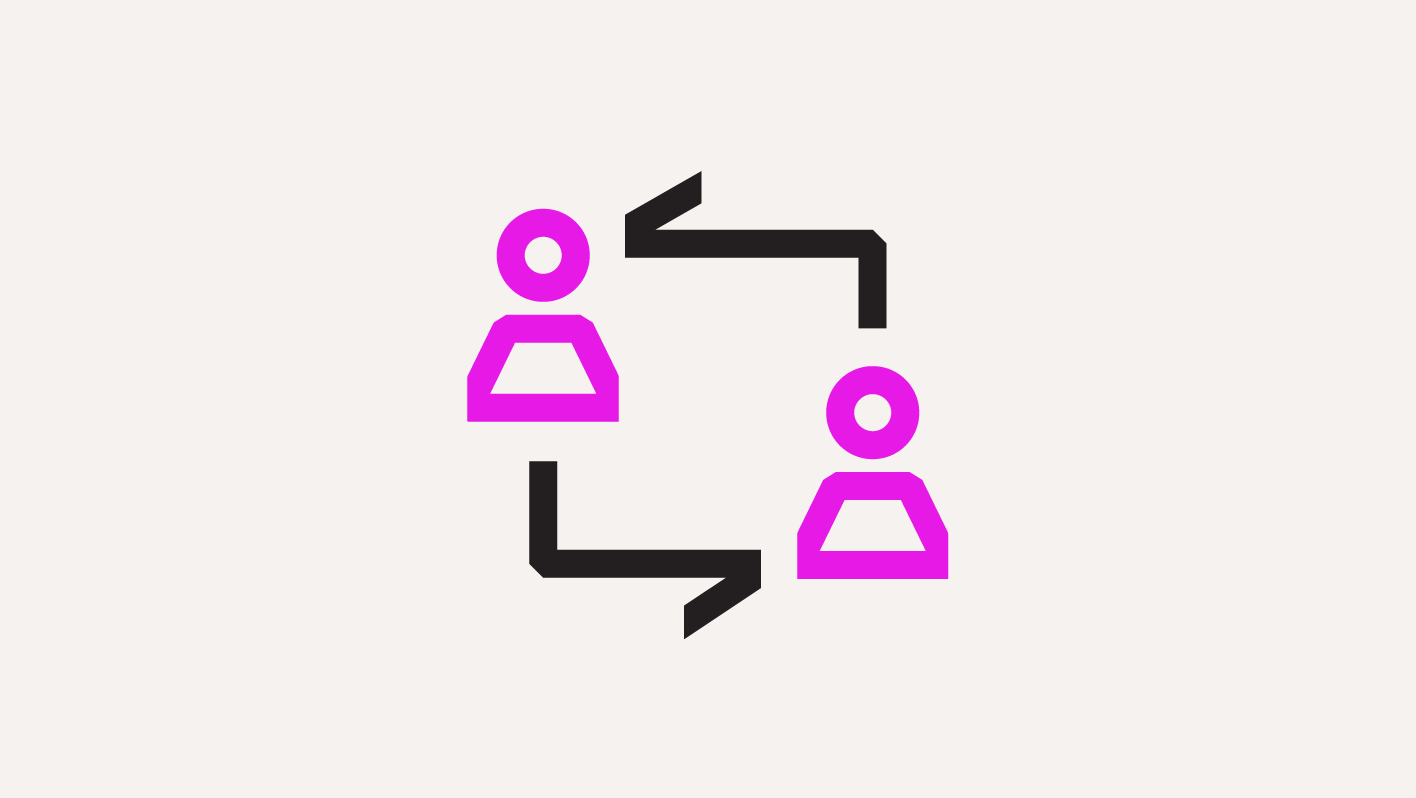
Build connections
Meet your peers and other industry experts, build your network and enjoy the evening social together - we consider it a highlight of the event.
Speakers
Lorem ipsum dolor sit amet, consectetur adipiscing elit. Nullam lobortis condimentum erat, ac aliquam justo vulputate eu.
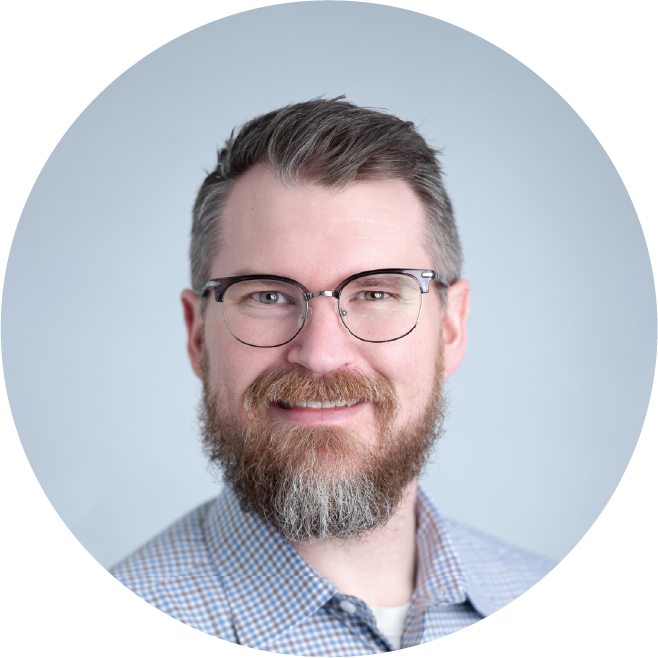
Name
Position
Presentation title
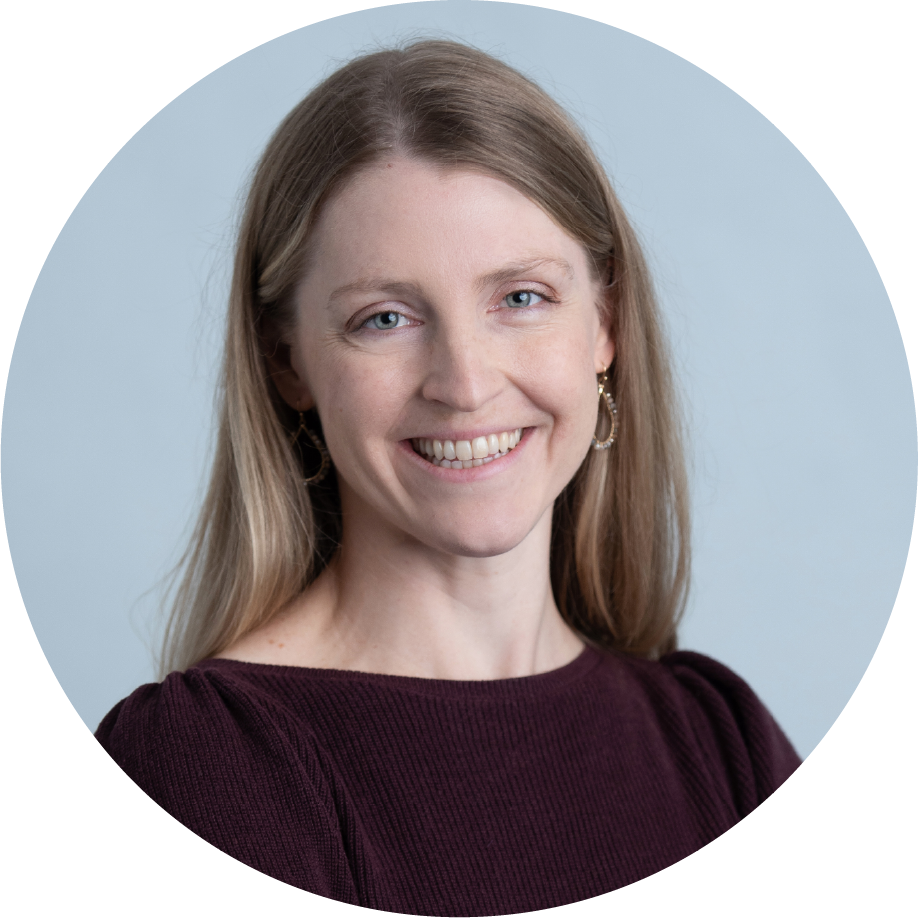
Name
Position
Presentation title
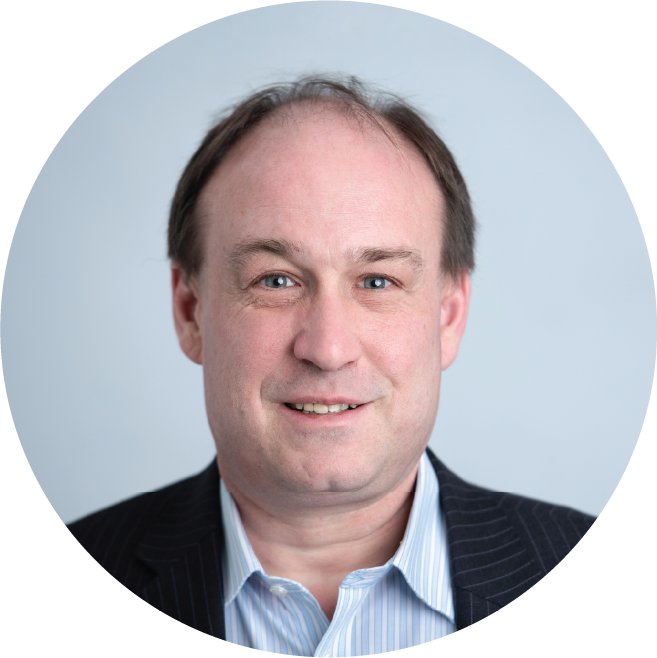
Name
Position
Presentation title
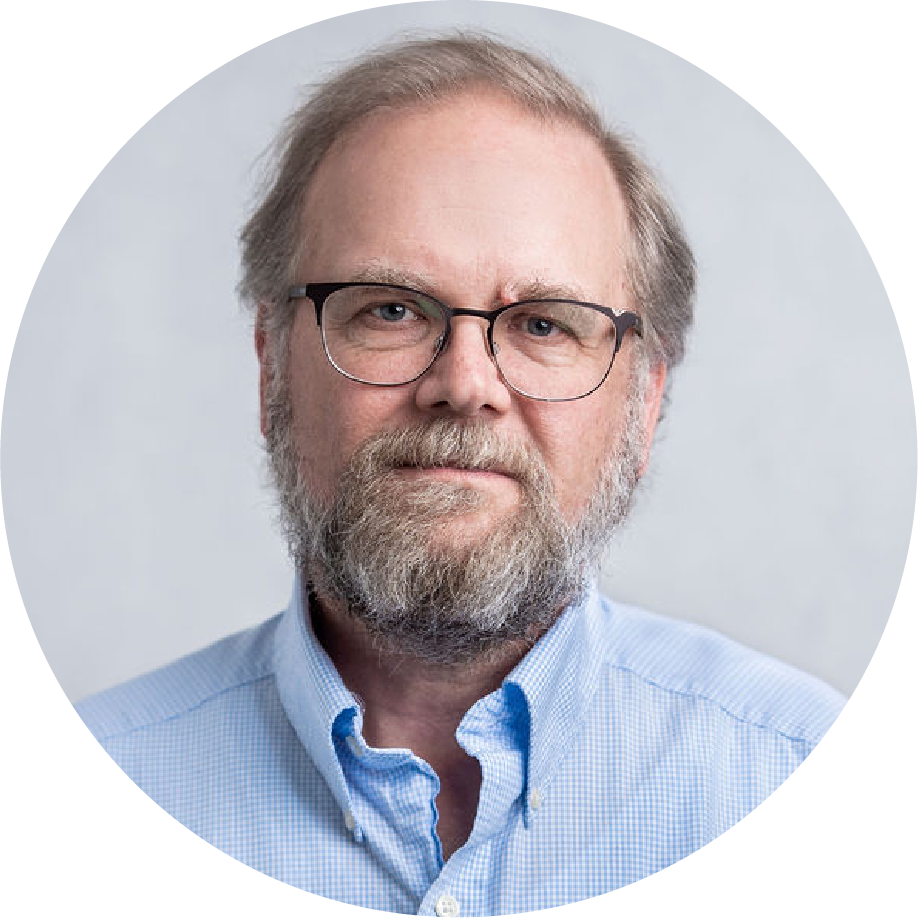
Name
Position
Presentation title
Jeremy Frey is a physical and digital chemist, interested in promoting interdisciplinary research across the physical and life sciences, from laser spectroscopy and imaging, computational modeling, and digital chemical duals.
He was the PI of the AI for Scientific Discovery Network and he is a co-I on the Physical Science Data Infrastructure project. For his most recent project, Jeremy is working with the Rosalind Franklin Institute to develop a laser driven high resolution soft x-ray microscope for biological imaging in the water window.
What is digital chemistry? How are automation and digitalization transforming the way we undertake chemical research? Have we been using AI to do this for ages or is it actually new?
I will discuss the general impact of AI, ML, and LLMs on chemical discovery and teaching. It is clear that the application of all these technologies depends on access to quality data, so I will focus on the different perceptions of FAIR data in industry and academia, describe how far we are along the journey to FAIR, and highlight some of the issues we have, taking examples from my own lab and computational research.
I will introduce some UK projects, namely the Physical Sciences Data Science Service (PSDS) and Physical Sciences Data Infrastructure (PSDI) and the AI Hubs (part of a FAIRground for physical sciences), to illustrate where we are in the physical sciences with respect to the investment in these areas for life sciences. “Smart Labs and Smart People”
Becky Upton was appointed as the Pistoia Alliance’s first female President in June 2022. She is a long-time supporter of pre-competitive collaboration in life sciences and healthcare R&D and the critical role it plays in advancing science and is passionate about diversity in STEM. Becky is responsible for leading the Pistoia Alliance’s strategy and defining its future within areas of increasing importance to the industry, such as data standards, emerging technologies, diversity and inclusion, sustainability, and precision medicine.
Becky first engaged with the Pistoia Alliance on its Lab of the Future project whilst at VWR (now part Avantor) where she worked for over a decade in sales, business development, and scientific services. From here, she joined the scientific instrumentation and analytical services company, Pion, as Managing Director, before moving to the Alliance as a project manager. She later became Chief Portfolio Officer and within this role established the Alliance’s first and thriving Diversity and Inclusion in STEM Leadership program. Becky re-joined the Alliance as President after a return to the commercial world as a Director at Impellam Group where she led the company’s STEM services strategy.
Becky has a PhD in Biochemistry from Imperial College and an MBA from Cranfield University.
The life sciences are fortunate to be rich in established ontologies, terminologies, and data models, for example MeSH, MedDRA, CDISC and the Human Phenotype Ontology. However, this richness presents its own challenges from the requirement to map between ontologies through managing multiple sources to choosing the right ontologies in a complex landscape. The ontology coverage is patchy, and for the pharma industry there are notable gaps that must be filled.
The Pistoia Alliance is a pre-competitive industry collaboration which aims to advance innovation through collaboration. One of its key strategic goals is delivering value from data of which shared ontology development is a key part.
In this presentation Becky Upton will describe the current and emerging data and ontology landscape and the part that Pistoia is playing in shaping the future. She will also examine how value is being derived from ontologies using knowledge-graphs and other approaches, 'AI-ready' data, and laboratory data collection.
Jessica Lanini joined the CADD group at Novartis Institutes for Biomedical Research (NIBR) as a Data Scientist in 2020. She supports MedChem project teams with machine learning and deep learning tools to accelerate DMTA cycle and compound prioritization. She has an MSc in Bioengineering from Campus Bio-medico of Rome since 2014, with a master thesis at ETH, Zurich, on robot control systems and human experiments.
She earned her PhD in Intelligent Systems and Robotics at EPFL, Lausanne, in 2019, focusing on data analysis, modeling and control of bipedal physical interaction while walking.
In this session, you will learn about synergies between academic and industry research when applying AI/ML for drug discovery. Both are essential for advancing molecular machine learning, but they often have different types and scales of studies, even though they share similar open research questions and long-term goals.
I will show you how machine learning models can speed up and enhance compound selection, covering the model life cycle: data preparation, model building, validation, and deployment. Also, I will talk about ways to apply models in the design-make-test-analyze cycle. I finish with suggestions to improve cooperation between academic and industrial institutions and further the progress of the field.
Timur Madzhidov is a Senior Product Manager for Reaxys at Elsevier. With expertise in chemoinformatics, he is a recognized researcher and educator who has co-authored over 70 scientific publications in the fields of reaction informatics, AI application in chemistry, algorithmic chemoinformatics, and chemistry-aware machine learning.
In his role at Elsevier, he leverages his experience in chemoinformatics and the application of artificial intelligence in chemistry to support the success of Reaxys users by delivering improved search and predictive capabilities, implementing new features, and enhancing the readiness of Reaxys data for AI and ML applications. Additionally, he collaborates with academic organizations to support Reaxys R&D efforts, which aim to advance reaction and molecular informatics by providing access to extensive chemistry data.
Prior to joining Elsevier, Timur held academic positions in cheminformatics and molecular modeling.
High-quality data and robust data processing and harmonization pipelines are essential when creating AI-driven predictive models. Such data pipelines enable aligning data from different sources thus facilitating development of accurate predictive models without having to create single data lakes.
However, different data sources may lack homogeneity in data representation, and some data representations are not suited for ML and AI modeling. Instead of breaking down data silos and creating a single data lake, we adopted the strategy of applying common preprocessing using unified data pipelines. This approach ensures consistency and comparability across datasets, resulting in harmonized, ML-ready datasets optimized for training models for predictive retrosynthesis and yield prediction.
I will describe my experience and best practices of such reaction data preprocessing.
In a recent customer project aimed at reducing synthesis failures in the lab, our goal was to create a predictive model capable of recommending favorable conditions for desired chemical transformations. We leveraged Elsevier's ML-optimized reaction dataset, compiled from published literature and patents, which primarily represents successful transformations with high yields. Consequently, we faced the challenge of underrepresentation of failed reactions. Such reactions can typically be found in company Electronic Laboratory Notebooks (ELNs) but are rarely covered in reaction collections extracted from publications.
By synchronizing these diverse datasets using our data preprocessing pipeline, we successfully unlocked the potential of disparate data sources. The result is a powerful predictive model capable of recommending optimal reaction conditions and reducing synthesis failures.
Josef Eiblmaier has more than two decades of successful commercial project and solutions experience in cheminformatics, life sciences, pharmaceuticals, biotechnology, and in the intellectual property sector. He currently heads the Discovery, Research, and Preclinical service line at PharmaLex, a Cencora company (formerly OSTHUS).
After completing his PhD in Technical Chemistry at the Technical University of Munich, Josef started his career at InfoChem, where he held various positions in marketing, business development, product development and management before joining OSTHUS in 2021.
New smart and data-driven technologies will help to improve success rates and efficiency in drug discovery. However, complex data-driven predictive models require careful assessment of both applicability and the quality of data. More than large amounts of data, good data quality is crucial for achieving the desired outcomes.
In many cases, data is distributed, in-homogeneous, and non-standardized, so the challenge is to efficiently merge, aggregate and harmonize heterogeneous data sets. In doing so, it is essential that the data is properly managed and governed.
In this talk, I'll showcase how data virtualization helps to solve the challenge of integrating heterogeneous drug discovery data from multiple sources and sites. A centralized data access platform capable of quickly integrating new data sources to speedy gain insights will be presented.
Karl-Heinz Baringhaus obtained his Ph.D. in synthetic organic chemistry at the University of Münster. After a postdoctoral fellowship at Stanford University he joined Hoechst AG in 1990 where he was working six years in Medicinal Chemistry. He then moved into Molecular Modeling and in 2000 became Head of Computational Chemistry at Aventis Pharma. From 2005 to 2010 he was Director of Drug Design at Sanofi-Aventis Pharma Deutschland GmbH. In 2010 he was promoted to Head of Structure, Design & Informatics consisting of Computational Biology & Bioinformatics, Computer-aided Drug Design, Scientific Computing & Data Management as well as Structural Biology. Since 2012, Karl-Heinz has been R&D Site Director in Frankfurt.
Drug Discovery and Development is facing high complexity and uncertainty, a low probability of success, long cycle times and costly investments. Challenges are for example, complex decisions across diverse disciplines, high volume of biological data, pressure on costs and efficiency as well as ethical and regulatory aspects.
Interdisciplinary integration of all disciplines and departments involved in drug discovery is mandatory. Genomic data from collaborations between industry and academia for example, helps understanding disease mechanisms and individual patient responses. Technologies like Computational Chemistry and Artificial Intelligence (AI) bridges handling and analysis of large and complex data, prediction of compound properties etc. In particular, the use of AI in modern drug discovery for identification, optimization and development of suitable molecules is indispensable.
Several examples from an industry perspective will be given on how to streamline drug discovery by better interdisciplinary collaboration, deeper insights, better decision filing as well as automation.
Nessa Carson received Master’s degrees in synthesis and catalysis from Oxford University and the University of Illinois at Urbana-Champaign.
She started her career as a synthetic chemist for AMRI, then moved within the company to run the high-throughput automation facility for Eli Lilly in Windlesham, working across discovery and process chemistry, then in high-throughput reaction optimization at Pfizer and then Syngenta. Nessa moved to AstraZeneca Early Chemical Development in 2022 as Digital Champion, focusing on digital transformation. She was awarded the Salters' Institute Centenary Award for early-career chemists with the potential to make an outstanding long-term contribution to industrial chemistry.
Modern chemistry is undergoing a transformative change with the advent of data and digitalization. The digitalization of chemistry involves tools including smart sensors, intelligent data aggregators, graphical interfaces for lab chemists to access computation, and laboratory robots, providing more and higher-quality data than ever before.
However, even open data can be difficult to use and access for chemistry practitioners who are not experienced in data science. Chemists need to be able to access enabling techniques through intuitive and user-friendly interfaces that save, not waste, time.
In this talk, I aim to explore solutions to this challenge through seamlessly combining fundamental chemical data sources with user experience in mind.
Peter Ertl studied organic chemistry and received his PhD at the University of Bratislava. Then he joined Ciba-Geigy in Basel as a molecular modeler. After a merger with Sandoz to form Novartis, he became Head of the Cheminformatics group in Pharma Research, responsible for development of new methods for calculation of molecular properties and cheminformatics web tools for medicinal chemists.
Peter is author of more than 130 publications covering all areas of cheminformatics and computational chemistry. His major research interests include analysis and visualization of chemical space, bioisosteric design and interactive web tools supporting the work of medicinal chemists.
In the cheminformatics community Peter is known as the author of several cheminformatics algorithms that have become industry standards, including a fast fragment based method to calculate molecular polar surface area and methods to calculate synthetic accessibility or natural-products likeness of molecules.
The majority of bioactive molecules contain a more or less complex ring system as a central structural element. This central core determines the shape of the molecule, keeps substituents in their proper positions and often also contributes to the biological activity of the whole system.
I will focus on the analysis of properties and structural features of ring systems in bioactive molecules and how they differ from properties of rings in common synthetic molecules. Our analysis revealed that the bioactive rings are distributed throughout the large portion of chemical space, but not uniformly; one can see several dense regions, where the bioactive rings often appear in small clusters, surrounded by empty regions. No simple set of descriptors separating active and inactive rings could be identified. The separation is best described by a neural network model taking into account a complex combination of many substructure features.
I will discuss the use of the results in the various drug discovery activities including library design, bioisosteric replacement and scaffold hopping.
Thrasyvoulos (Thras) Karydis is the CTO at DeepCure, an AI-driven biotechnology company focusing on inflammation and autoimmune diseases. Thras is leading the development of DeepCure's groundbreaking AI platform, and he is a thought leader on computational tools and software for small molecule design and automated chemistry.
He is an alumni of the Molecular Machines of MIT Media Lab and the MIT Center for Bits and Atoms.
When binding data for a target is not available, limited, or biased, most AI drug discovery companies are ill-equipped to deliver novel, viable starting points for optimization and/or leads. This is especially true for therapeutic targets in inflammation, where targeting Protein-Protein and Protein-DNA interactions requires working with highly-charged, highly-3D-shaped molecules, pushing the limits of existing cheminformatics and computational chemistry tooling. Moreover, in inflammation there is a narrow window of acceptability for ADME-tox properties due to chronic dosing dictating the need for multi-dimensional analysis of experimental data.
To tackle these challenges, biases need to be removed from every part of the design-build-test-learn cycle. At DeepCure we are removing limitations to novelty and diversity in each step, with AI tools that give a framework for identifying novel binding opportunities and design tools that allow drug design in novel chemical space while taking into account desired ADME-tox profile and synthesizability requirements. Accessing novel chemical space is made possible by a robotic automated custom synthesis system that allows for the reproducible multi-step synthesis of molecules from 100+ different reaction types.
Adrian Stevens joined Chemaxon as Chief Product Officer in 2022. Adrian has spent approximately 15 years working in Life Sciences software companies, in product management roles for most of his time.
As a computational chemist at BioFocus PLC, over the course of nearly 10 years, he supported a wide range of areas in therapeutic research, from oncology, metabolic diseases, autoimmune and pain-based medicines. During this time, he specialized in designing small molecule drugs that targeted protein kinases and GPCR proteins, leading to a number of scientific publications and patents.
Adrian has a PhD in Computational Chemistry from the University of Portsmouth.
I will showcase new approaches and ideas that together illustrate potential avenues of exploration within Chemaxon’s portfolio. This will be an opportunity for you to gain insights into how our solutions enhance data integration, streamline workflows, and facilitate collaboration across research teams. This talk is not only a showcase of our latest advancements but also an open invitation to engage with us and help bridge the silos within drug discovery.
