Introduction
In pharma R&D, year after year billions of dollars are spent on projects that will never result in a marketed drug. Behind every successful approval, there are, on average, nine other candidate molecules that have failed in clinical trials, plus an untold number of obstacles, pitfalls, and uncertainties, all contributing to the extremely high development costs. Despite extensive preclinical testing, potential drugs may not produce the desired therapeutic effects in humans, or there might be some safety issues, thus promising compounds can fail in the latest, most expensive phase of an R&D project.
Decreasing failure rate by designing even better leads and clinical candidates has been a long-existing goal of every player in drug discovery. From the many factors that support this goal, here we would like to highlight two: unrestrained collaboration in and between research teams and consistent consideration of all required understanding, perspectives and insights when reviewing compounds and making a decision on “what to make next” in the hit to lead and lead optimization phases.
In the background, both these factors are deeply connected to the data that can be accessed and used by research team members. In a classic, hypothesis-driven discovery project, where potential drug candidates are optimized through a series of design-make-test-analyze (DMTA) cycles, a diverse landscape of new data is generated in each phase of the cycle. Ideally, all of this data, along with similar information from parallel (or legacy) projects are available for the entire project team during the whole course of the project. What we see, more often than not, is a fragmented data landscape where access to information can be blocked by disconnected software tools, incompatible legacy systems, differing file formats or too rigid company policies. Such fragmentation and the data silos emerging in its wake are among the biggest challenges of data management in pharma R&D. But what does it mean in practice? How do silos hamper the work of drug discovery teams? And how can we break them down?
Data silos in the DMTA stages
The risk of data getting locked in silos naturally increases with the number of data sources, as well as with the number of applications, and the number of people generating or using data in an organization. As drug discovery workflows are increasing in complexity and becoming more data-heavy in all stages of the design-make-test-analyze process, we have to expect an increasing probability of the pharma R&D data landscape becoming even more fragmented, unless we proactively address the underlying root causes. Without attempting to be comprehensive, we would like to use the following examples to demonstrate how data silos can appear in the course of a DMTA iteration.
Design
The Design phase, where new compound ideas are born, is traditionally one of the least standardized stages of drug discovery. Even with the advent of generative AI and other ML-based methods, medicinal chemists’ expertise and intuition are still among the most important driving forces behind drug discovery innovation. People in such a role often work individually on their molecule designs and keep refining them before they are ready to share their best ideas with their team. During their solo work, they use a series of software tools to generate new chemical structures, as well as to predict their properties or screen them virtually. While working with a number of different applications and having a private workspace for chemists to ideate on their own do not inevitably have to lead to data silos, current practices show that it is still happening more often than not.
-
- Part of the reason is that even today, several computational chemistry tools - used for structure generation, property prediction or virtual screening - are not connected to each other and are not integrated into the wider R&D IT framework either. Users of such tools usually rely on file export-import - or custom-built workflows using KNIME or a similar tool - to move the generated or screened structures to a virtual compound repository or to some other software, a step which can be made unnecessarily difficult by proprietary file formats and other incompatibilities between applications. Not to mention that such exported results typically include the structures themselves, but not the parameters and other metadata used to generate and select them, which means that a large part of the design context will be lost for future review.
- Earlier, we wrote about what kind of difficulties R&D teams have to face by using static files, such as Microsoft Excel and PowerPoint, to document design hypotheses, and research findings, or to store chemical structures with respective assay results. The most conspicuous effect of locking chemical structures, design related considerations and decisions into such disconnected sources is how painful it becomes to update and search in that data. Teams have to go through the tedious process of versioning offline files, keeping track of where they can find the latest version and making sure that updated versions are shared with all interested parties in time. Moreover, even the simplest tasks, such as checking if the same or similar compound ideas have already been designed by others will take significant effort and add several extra hours to an already lengthy and complex process.
- Besides the difficulties of accessing up-to-date information in an ongoing project, segmented research data has a much longer lasting effect as well. It can make an organization’s design history - collected over several years and dozens of research projects by hundreds of scientists - practically completely hidden from the current team’s view, making it impossible for them to rely on the company-wide knowledge accumulated over past decades.
- Often, it is not only the design team who needs to access design related data. Chemical structures, synthesis plans, and priorities are all part of the information which is regularly shared with internal collaborators, such as synthetic chemistry and pharmacology teams, or external partners and CROs. Without an effective, secure, and trusted data sharing process, collaborators’ objectives can easily get misaligned, which may lead to avoidable delays or wasted time due to duplicated work. For example, an external synthesis partner who does not get notified when internal compound priorities change, may spend additional days in the lab working on already down-prioritized compounds instead of focusing on the most important ones. The lack of built-in security features can be the source of other types of delays as well. For example, without a granular access control mechanism, when people want to share data externally, they have no other choice but to manually check and re-check that no data will be shared that is not to be shared. One such event might take only a couple of minutes, but dozens of people having to do it on a weekly or daily basis can add up to a considerable amount of time.
Make
The Make phase, also known as the Synthesis phase, is where the designed compounds are produced in the lab. From the aspect of data management, it might seem more straightforward; however, data silos and inefficient flow of communication can cause significant problems here as well. Let’s see a few potential pitfalls.
-
- A possible problem can be that synthesis data and methods are not transparent or easily accessible. For example, suppose a chemist is unaware that a particular synthesis process has already been optimized by someone else in the company. In that case, they might spend time and resources redoing the same (or very similar) work. The reason for this can be e.g. a chemically non-aware ELN system, where the ability to search for similar reactions or products is not possible. Redundancy slows down the feedback loop to the design team, delaying the project's progress.
- The format of the data the synthetic team receives from the design team is also a crucial factor since molecules have to be interpreted in the same way in all teams. The design team might use specialized software for molecular modeling, while the synthesis team uses other tools for planning and executing chemical reactions. If these tools are not integrated, transferring data between them can be a cumbersome process. Data might need to be exported and imported or even manually re-entered, increasing the risk of errors. For example, the different interpretation of stereochemistry in the case of using different file formats and/or applications is a recurring problem, leading to the synthesis process producing incorrect results.
- During the synthesis phase, a lot of new data is generated, including detailed records of the chemical reactions, yields, and any issues encountered during the process. When new compounds are synthesized, the knowledge gained during this process is invaluable for future projects. However, if the data from the synthesis phase is not properly integrated and stored in a central repository, this knowledge can be lost. Future teams might not have access to insights on what worked and what didn’t, leading them to repeat the same mistakes (getting us back to the first bullet point). Molecular structures, reactions, and the corresponding data are frequently shared through slide decks or email, making it nearly impossible to effectively search through past data.
- In a well-integrated data environment, the progress of synthesis can be tracked in real time, and any issues can be quickly identified and addressed. If updates on synthesis progress are stored in a siloed environment or communicated through inconsistent channels (e.g., via email), project managers might not have a clear view of how the project is advancing. When there are delays in synthesis, project leadership may struggle to realize any existing bottlenecks and pinpoint the root cause due to fragmented data, making it harder to implement timely and effective solutions next time (e.g., to decide whether it is still feasible to synthesize the desired compound or if they should pivot to a different one).
- Data fragmentation may lead to cumbersome project tracking and belated updates during the analysis and quality control of the synthesized compounds as well. Not only because the teams responsible for these processes typically work quite separately from the rest of the research team, but they also rely on data management solutions, i.e., LIMS, that are oftentimes very much disconnected from the rest of the R&D IT infrastructure.
Test
- Similarly as in the case of the Synthesis phase, assay data often comes from multiple sources and platforms, each generating different types of data. If this data is stored in separate, unconnected systems, it can be challenging to get a comprehensive view of a compound's performance. For example, biological activity data might be in one database, toxicity data in another, and pharmacokinetic data in yet another.
- Assay results might be reported in various formats depending on the source, including spreadsheets, databases. Inconsistent data formats and the lack of a well-designed extract-transform-load (ETL) system can make it hard to integrate and analyze the data effectively. Scientists might spend excessive time converting and cleaning data rather than focusing on analysis.
- When the biology team works in isolation, assay results are often not shared effectively and promptly or are not stored in the same system that houses hypotheses and design sets. This can lead to inefficient design iterations, as the design team might continue to produce compounds that have already been found to be less effective. In many organizations, assay results are not immediately accessible to the entire project team. Data might be held by specific individuals or departments, leading to delays in sharing results with the design and synthesis teams. This lag can slow down the iterative process of the DMTA cycle, delaying critical decisions on which compounds to advance or modify. Timely access to assay data is essential for maintaining the momentum of the drug discovery process.
The Test phase is about evaluating the biological activity, potency, and selectivity of the synthesized compounds.
Analyze
- The Analysis phase is where the data generated from the design, make, and test phases is thoroughly examined to draw meaningful conclusions about the compounds. This phase involves interpreting assay results, understanding structure-activity relationships (SAR), and deciding on the next steps. As this is the step when researchers need all the data to form a complete picture, it is the most exposed to data silos.
- If predicted parameters, synthesis details, and assay results are stored in separate databases, significant time and effort might be spent on data conversion and cleaning.
- If the SAR analysis data is conducted in a separate application, it can prevent a comprehensive understanding of data trends and the identification of promising candidates. If the shared data is not interactive, users in the design team may not have access to manipulate and in-depth explore the SAR data to uncover new insights or correlations.
- Advanced analytical tools and algorithms (e.g. machine learning models) are essential for deriving insights from complex datasets. However, if SAR analysis data is fragmented across different systems, these tools cannot be fully leveraged.
Although this is not an exhaustive list, the examples above can definitely outline the circumstances frequently leading to the formation of silos with fragmented research data. From a technical perspective, among the most typical reasons are the lack of integration, decentralized data storage and incompatible file formats. The root causes, however, usually run even deeper, and are connected to the lack of organizational best practices to capture, share and access data on the one hand, and security related concerns on the other. When different departments, who have to work together, fail to synchronize their processes and the software they use, the risk of building silos can easily multiply. Add to that the tendency of outsourcing more and more tasks to CROs and other external collaborators, and we will immediately see why it is so difficult to avoid data silos in such a multiplayer domain as drug discovery.
Are data silos simply inevitable?
In the previous section, we offered a glimpse into the many ways how data silos can be formed in the everyday work of a pharma R&D organization. On the positive side, we can note that project meetings in an R&D setup typically involve participants from both the chemistry and the biology departments, who actively share data with each other via presentations. While it is not ideal, it can partially compensate for the negative effect of data silos.
But are those silos really inescapable? Instead of building barriers that segment research data, introducing expert systems facilitating integration and offering a centralized R&D data repository can minimize the risk of data silos. Good quality integration streamlines capturing and harmonizing information from the vast network of software tools used in drug discovery without the need to regularly copy-paste or export, convert and import data sets. Storing the captured and harmonized information in a centralized repository helps eliminate duplications and ambiguity in the research data, and it also allows analyzing all those insights from a single platform making it possible for the R&D team to capitalize on legacy information and knowledge from the entire organization. Having a fine-grained access control system in place means that security does not have to be sacrificed even when data has to be accessed by external collaborators. Last but not least, any expert system has to come with a high-level of flexibility to support the diverse workflows and preferences of the users, otherwise they might give up on the new software and return to their old ways resulting in even more data silos.
What is your story? How did you manage to decrease data fragmentation? What kind of silos are you fighting now?
References
“44% of the survey sample saw increasing the use of cloud/SaaS solutions as being among the three most important steps their company is taking to improve operational agility and keep up with market demands over the next 3-5 years.”
https://www.innoplexus.com/blog/breaking-down-data-silos-to-revolutionize-pharmaceutical-industry/
https://www.anjusoftware.com/insights/data-science/data-silos/
“One way of breaking down the barriers [caused by data silos] is by deploying products that utilise a common data ecosystem enabling real-time data access,”“Regardless of the size or scope of the challenge, it’s unlikely that any organization will find a one-size-fits-all solution. Rather, life sciences companies are likely to work with a number of digital tools and vendors to realize their goals.” Integrability
Did you find this article useful?
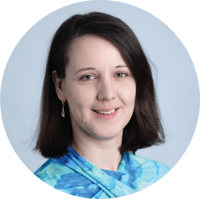
Later, she joined the Application Scientist team of Chemaxon where she is responsible for supporting clients in designing cheminformatics solutions and for conducting application studies.
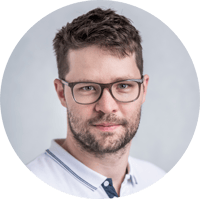